Video generation model based on text description information and generative adversarial network
A technology for describing information and generating models, applied in the field of computer vision, can solve the problems of lack of generalization, difficult training of GAN, inflexibility, etc., and achieve the effect of good generalization ability
- Summary
- Abstract
- Description
- Claims
- Application Information
AI Technical Summary
Problems solved by technology
Method used
Image
Examples
Embodiment 1
[0023] The present invention mainly includes two parts: text information processing and generative confrontation model design, corresponding to figure 1 The parts indicated by (1) and (2) in.
[0024] The first is the effective processing of text information. The main purpose is to obtain word vectors that are closely related to videos and have generalization properties. The present invention refers to the objects2action model proposed by Mihir Jain et al. figure 2 shown. The model uses an image data set with text information (usually label information, not a single word, usually 2 to 4 words, such as: brush hair, diving springboard 3m, etc.) as a training set, expressed as D≡{x,y }, where x is the image and y is the label information; the video with label information is used as the test set, expressed as T≡{v,z}, where v is the video and z is the video label information. And y and z are completely disjoint.
[0025] like figure 2 As shown, first, the model will use the ...
PUM
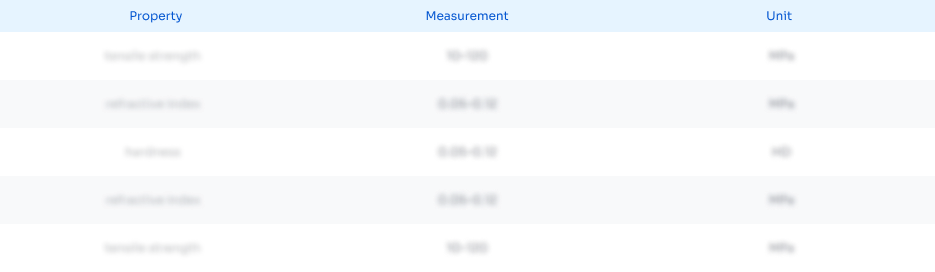
Abstract
Description
Claims
Application Information
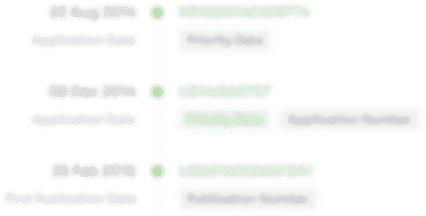
- R&D Engineer
- R&D Manager
- IP Professional
- Industry Leading Data Capabilities
- Powerful AI technology
- Patent DNA Extraction
Browse by: Latest US Patents, China's latest patents, Technical Efficacy Thesaurus, Application Domain, Technology Topic, Popular Technical Reports.
© 2024 PatSnap. All rights reserved.Legal|Privacy policy|Modern Slavery Act Transparency Statement|Sitemap|About US| Contact US: help@patsnap.com