Generative adversarial network-based grayscale picture colorizing method
A colorization and network technology, applied in the field of deep learning and image generation, can solve the problems of easy loss of details and complex operation of images
- Summary
- Abstract
- Description
- Claims
- Application Information
AI Technical Summary
Problems solved by technology
Method used
Image
Examples
Embodiment Construction
[0066] The picture generation method combining DiscoGAN, Progressive Growing GAN, WGAN and CGAN is explained in detail below in conjunction with the accompanying drawings.
[0067] The grayscale image colorization system of the present invention should include the following parts: sample data collection, sample image preprocessing, generation of adversarial network model establishment, network training and testing and adjusting hyperparameters. The main steps included in the present invention are: collecting pictures and performing preprocessing, inputting the pictures into the generative confrontation network, training the survival confrontation network, adjusting the hyperparameters of the generative confrontation network and repeated training to obtain the final model, such as figure 1 shown. Its system structure is as figure 2 shown. The sample data collection link is responsible for collecting enough grayscale and color pictures that contain rich detailed information a...
PUM
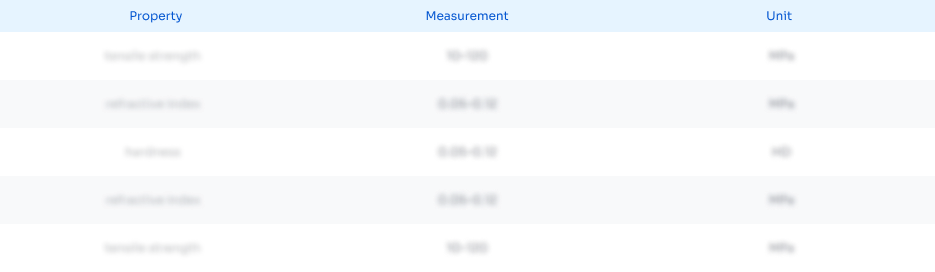
Abstract
Description
Claims
Application Information
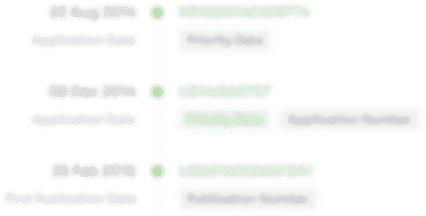
- R&D
- Intellectual Property
- Life Sciences
- Materials
- Tech Scout
- Unparalleled Data Quality
- Higher Quality Content
- 60% Fewer Hallucinations
Browse by: Latest US Patents, China's latest patents, Technical Efficacy Thesaurus, Application Domain, Technology Topic, Popular Technical Reports.
© 2025 PatSnap. All rights reserved.Legal|Privacy policy|Modern Slavery Act Transparency Statement|Sitemap|About US| Contact US: help@patsnap.com