Remote sensing image scene classification method based on deep convolutional neural network and multi-kernel learning
A convolutional neural network and multi-core learning technology, applied in character and pattern recognition, instruments, computing, etc., can solve the problems of difficulty in selecting classifier parameters, redundancy, and incomplete coverage of feature information, so as to optimize the classification process, strengthen the Expressive and robust effects
- Summary
- Abstract
- Description
- Claims
- Application Information
AI Technical Summary
Problems solved by technology
Method used
Image
Examples
Embodiment Construction
[0062] The technical solutions of the present invention will be further described below in conjunction with the accompanying drawings and embodiments.
[0063] Such as figure 1 Shown, technical scheme of the present invention is described in further detail as follows:
[0064] (1) Use the deep convolutional neural network to train the remote sensing scene image, and use the output of the two fully connected layers learned as the features of the remote sensing scene image. These features include the underlying features of the remote sensing scene image. Such features are obtained through The middle-level features obtained by the front-end convolutional layer of the deep convolutional neural network, such features are obtained through the middle convolutional layer of the deep convolutional neural network, and high-level features, such features are obtained through the back-end convolution of the deep convolutional neural network layer obtained.
[0065] (1.1) Construct remote...
PUM
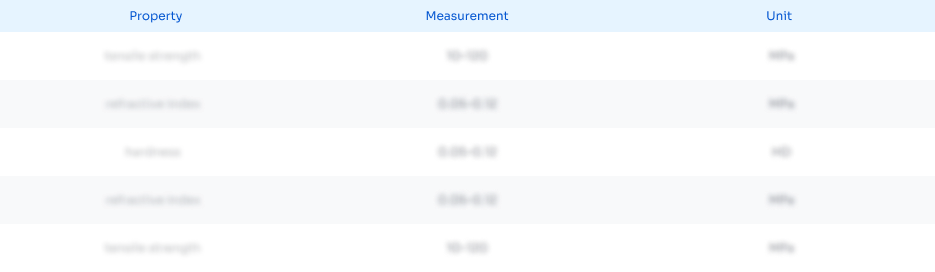
Abstract
Description
Claims
Application Information
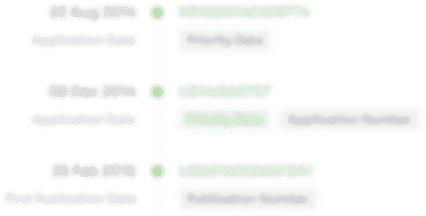
- R&D Engineer
- R&D Manager
- IP Professional
- Industry Leading Data Capabilities
- Powerful AI technology
- Patent DNA Extraction
Browse by: Latest US Patents, China's latest patents, Technical Efficacy Thesaurus, Application Domain, Technology Topic, Popular Technical Reports.
© 2024 PatSnap. All rights reserved.Legal|Privacy policy|Modern Slavery Act Transparency Statement|Sitemap|About US| Contact US: help@patsnap.com