Dual-sampling integration classification model based on Fisher kernel
A double-sampling and classification model technology, applied in the field of pattern recognition, to achieve accurate and improved classification effects
- Summary
- Abstract
- Description
- Claims
- Application Information
AI Technical Summary
Problems solved by technology
Method used
Image
Examples
Embodiment Construction
[0010] Below in conjunction with accompanying drawing and example the present invention will be further introduced: the system designed by the present invention is divided into four modules altogether.
[0011] Part 1: Data Preprocessing
[0012] The number of sample subsets generated by sampling corresponds to the number of sub-classifiers, and the sub-classifiers are trained on the corresponding subsets. Define the negative class as the majority class sample, and the positive class as the minority class sample. All training samples are synthesized into a training matrix X according to the rule that each column is a sample for storage.
[0013] Part II: Fisher Kernel Mapping
[0014] In this part, the samples in the Fisher kernel space are used to form a new sample set. Therefore, in order to construct the Fisher kernel map, it is necessary to use the EM algorithm to obtain the component parameters of the Gaussian mixture model (GMM) for the data set. Now suppose the origi...
PUM
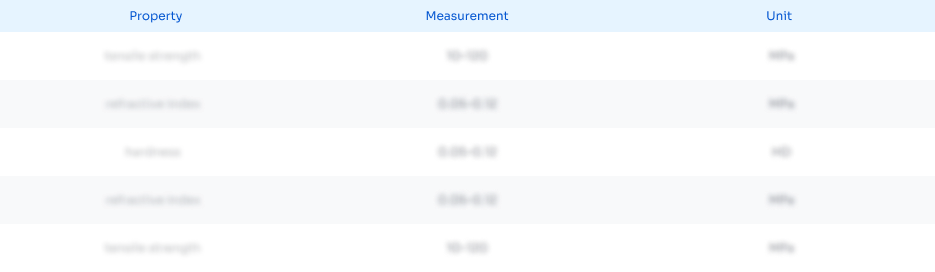
Abstract
Description
Claims
Application Information
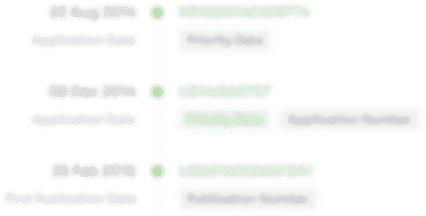
- R&D
- Intellectual Property
- Life Sciences
- Materials
- Tech Scout
- Unparalleled Data Quality
- Higher Quality Content
- 60% Fewer Hallucinations
Browse by: Latest US Patents, China's latest patents, Technical Efficacy Thesaurus, Application Domain, Technology Topic, Popular Technical Reports.
© 2025 PatSnap. All rights reserved.Legal|Privacy policy|Modern Slavery Act Transparency Statement|Sitemap|About US| Contact US: help@patsnap.com