Multi-empirical kernel classifier based on Universum learning
A technology of kernel classification and experience, which is applied in the field of multi-experience kernel learning mechanism, can solve problems such as the ineffective combination of Universum learning and multi-experience kernel, and achieve the effect of solving the imbalance problem
- Summary
- Abstract
- Description
- Claims
- Application Information
AI Technical Summary
Problems solved by technology
Method used
Image
Examples
Embodiment Construction
[0011] Below in conjunction with accompanying drawing and table, the present invention is further introduced: the present invention is divided into four steps altogether. Suppose there is dataset X: where x i ∈ R d ,and For sample set X represents an N×d sample matrix, each row represents a sample, and d represents the dimension of the sample.
[0012] Part 1: Multi-empirical kernel mapping: Multi-empirical kernel mapping refers to passing the original data sample X through m empirical kernel functions Φ e Mapped to the corresponding m new feature spaces The feature space dimension after each mapping is ne.
[0013] The second part: Generate Universum samples: In the mapped feature space, use the mapped sample data to generate Universum samples. Here, the present invention introduces a new Universum sample generation method IMU. It is defined as follows:
[0014]
[0015] N p Indicates the number of positive samples, N n Indicates the number of negative sampl...
PUM
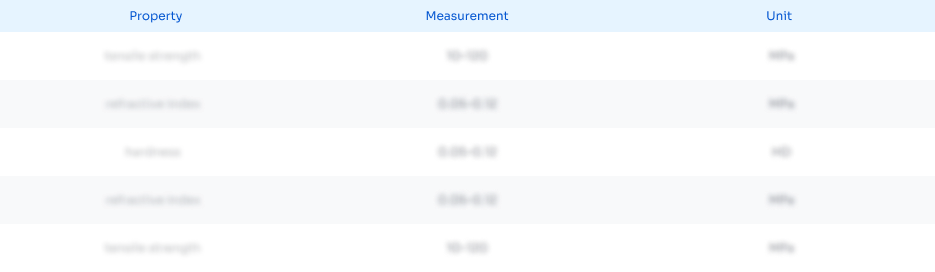
Abstract
Description
Claims
Application Information
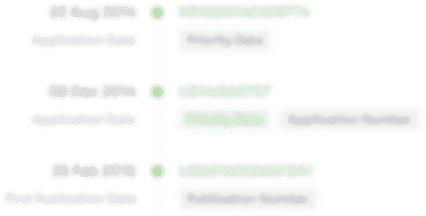
- R&D Engineer
- R&D Manager
- IP Professional
- Industry Leading Data Capabilities
- Powerful AI technology
- Patent DNA Extraction
Browse by: Latest US Patents, China's latest patents, Technical Efficacy Thesaurus, Application Domain, Technology Topic, Popular Technical Reports.
© 2024 PatSnap. All rights reserved.Legal|Privacy policy|Modern Slavery Act Transparency Statement|Sitemap|About US| Contact US: help@patsnap.com