Transformer fault type diagnosis method based on semi-supervised DBNC
A technology of transformer faults and diagnosis methods, applied in the fields of instruments, measuring electrical variables, biological neural network models, etc.
- Summary
- Abstract
- Description
- Claims
- Application Information
AI Technical Summary
Problems solved by technology
Method used
Image
Examples
Embodiment Construction
[0029] The invention proposes to use the DBNC network to select samples with high confidence and expand the number of training samples.
[0030] Deep Belief Network Classifier
[0031] The network structure of the deep belief network classifier is composed of an input layer, several Restricted Boltzmann Machines (RBM) and a top classification layer. The top classifier is a Softmax classifier, which is characterized by While giving the classification results, it also gives the probability of each result, which is very suitable for solving nonlinear multi-classification problems.
[0032] When the deep belief network classifier deals with multi-classification problems, its training process is divided into two stages: pre-training and tuning.
[0033] (1) In the pre-training stage, the layer-by-layer training method is used to initialize the connection weights and offsets between each layer of the network. This process is an unsupervised learning process.
[0034] Taking a sing...
PUM
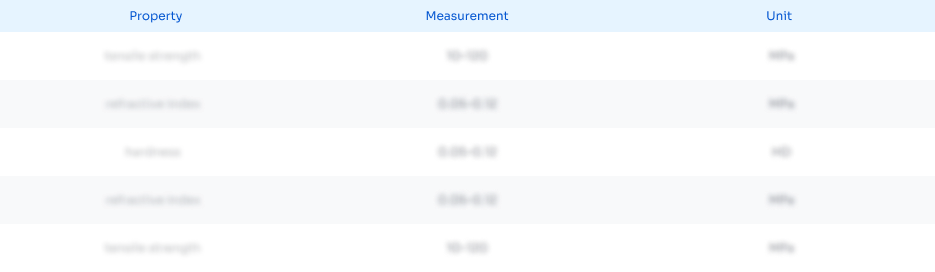
Abstract
Description
Claims
Application Information
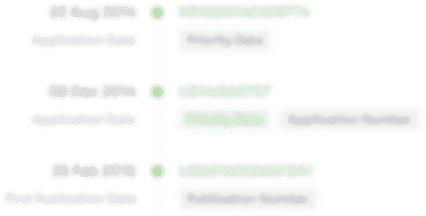
- R&D Engineer
- R&D Manager
- IP Professional
- Industry Leading Data Capabilities
- Powerful AI technology
- Patent DNA Extraction
Browse by: Latest US Patents, China's latest patents, Technical Efficacy Thesaurus, Application Domain, Technology Topic, Popular Technical Reports.
© 2024 PatSnap. All rights reserved.Legal|Privacy policy|Modern Slavery Act Transparency Statement|Sitemap|About US| Contact US: help@patsnap.com