Laplace spare deep belief network image classification method
A deep belief network, image technology, applied in the fields of deep learning and image processing
- Summary
- Abstract
- Description
- Claims
- Application Information
AI Technical Summary
Problems solved by technology
Method used
Image
Examples
Embodiment 1
[0079] Such as figure 1 As shown, a sparse deep belief network image classification method based on Laplace function constraints, the specific steps are as follows:
[0080] Step 1. Select an appropriate training image data set, and perform image preprocessing on it to obtain a training data set.
[0081] Since the image classification focuses on the feature extraction process, the color image is converted into a grayscale image through binarization, and the grayscale value is normalized to [0,1], so that only a two-dimensional grayscale The degree-level matrix is used for feature extraction. The specific normalization formula is as follows:
[0082]
[0083] in, is the feature value of the image dataset, x max and x min are the maximum and minimum values of all features of the image dataset, respectively, and x is the normalized image dataset.
[0084] Step 2. Use the preprocessed training data set for pre-training of the LSDBN network model. According to the i...
Embodiment 2
[0156] Example 2: Experiments on the MNIST handwriting database
[0157] The MNIST handwriting data set includes 60,000 training samples and 10,000 test samples, and the size of each picture is 28*28 pixels. In order to facilitate the extraction of image features, the present invention extracts different numbers of images of each category from 60,000 training data for experimental analysis. Among them, the model includes 784 visible layer nodes and 500 hidden layer nodes, the learning rate is set to 1, the batch size is 100, the maximum number of iterations is 100, and the CD algorithm with a step size of 1 is used to train the model.
[0158] Table 1 shows the sparsity measurement results on the MNIST data set in the present invention, and a comparative analysis with the other two sparse models. The sparsity measurement method is as follows:
[0159]
[0160] For sparse models, the higher the sparsity, the higher the algorithm stability and the stronger the robustness. ...
Embodiment 3
[0169] Example 3: Experiments on the Pendigits Handwriting Recognition Dataset
[0170] The Pen-Based Recognition of Handwritten Digits (PenDigits) data set includes 10992 data samples, which are divided into 10 categories, including 7494 training data, 3298 test data, and 16 feature vectors for each sample. Also, different numbers for each class images for analysis. Set the visible layer nodes to 16, the hidden layer nodes to 10, the learning rate to 1, the batch size to 100, and the maximum number of iterations to 1000.
[0171] figure 2 It shows the classification accuracy results of LS-RBM in the present invention on the Pendigits handwriting recognition data set based on the number of different samples of each class. It can be seen that when the number of samples of each class is larger for most algorithms, the classification accuracy rate is also getting higher and higher. high. The LS-RBM algorithm still achieves the best classification accuracy on the PenDigits dat...
PUM
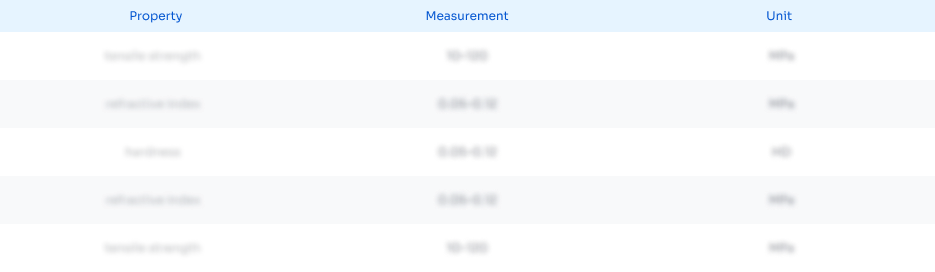
Abstract
Description
Claims
Application Information
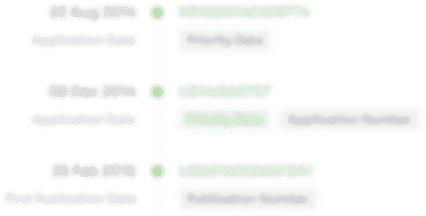
- R&D Engineer
- R&D Manager
- IP Professional
- Industry Leading Data Capabilities
- Powerful AI technology
- Patent DNA Extraction
Browse by: Latest US Patents, China's latest patents, Technical Efficacy Thesaurus, Application Domain, Technology Topic, Popular Technical Reports.
© 2024 PatSnap. All rights reserved.Legal|Privacy policy|Modern Slavery Act Transparency Statement|Sitemap|About US| Contact US: help@patsnap.com