Image classification method based on structure optimization sparse convolutional neural network and medium
A convolutional neural network and classification method technology, applied in the field of model structure sparse and image classification, can solve the problem of no measurement standard, model classification effect will not bring any contribution, large overhead, etc., to achieve the effect of high computational complexity
- Summary
- Abstract
- Description
- Claims
- Application Information
AI Technical Summary
Problems solved by technology
Method used
Image
Examples
Embodiment Construction
[0047] The technical solutions in the embodiments of the present invention will be described clearly and in detail below with reference to the drawings in the embodiments of the present invention. The described embodiments are only some of the embodiments of the invention.
[0048] The technical scheme that the present invention solves the problems of the technologies described above is:
[0049] Such as figure 1 As shown, the image classification method based on the structurally optimized sparse convolutional neural network provided in this embodiment includes the following steps:
[0050] Step 1: Take the minimization of the cross entropy function between the predicted value and the real label as the optimization goal, use the training set sample as the input, use the Adam algorithm as the optimization algorithm, set the learning rate to 0.001, and pre-train a LeNet-5 convolutional neural network network until the model reaches convergence on the training set, and save the...
PUM
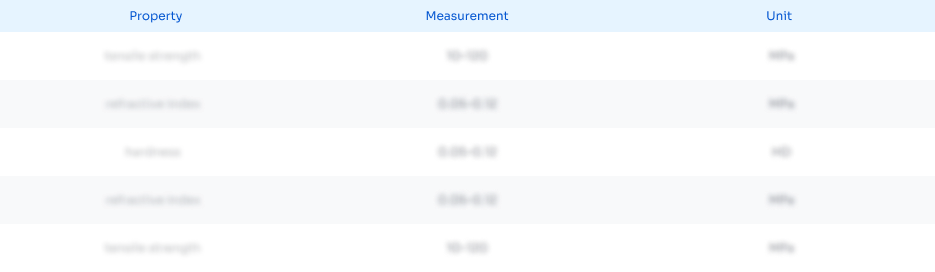
Abstract
Description
Claims
Application Information
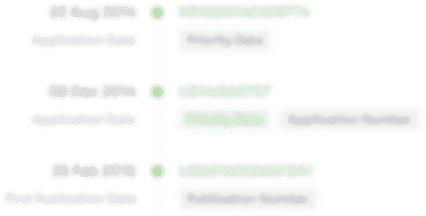
- R&D
- Intellectual Property
- Life Sciences
- Materials
- Tech Scout
- Unparalleled Data Quality
- Higher Quality Content
- 60% Fewer Hallucinations
Browse by: Latest US Patents, China's latest patents, Technical Efficacy Thesaurus, Application Domain, Technology Topic, Popular Technical Reports.
© 2025 PatSnap. All rights reserved.Legal|Privacy policy|Modern Slavery Act Transparency Statement|Sitemap|About US| Contact US: help@patsnap.com