Emotion electroencephalogram signal classification method based on cross-connection type convolutional neural network
A convolutional neural network and EEG signal technology, applied in the field of deep learning classification of emotional EEG signals, can solve the problem of less recognition of EEG signals
- Summary
- Abstract
- Description
- Claims
- Application Information
AI Technical Summary
Problems solved by technology
Method used
Image
Examples
Embodiment Construction
[0052] Such as figure 1 As shown, this example includes the following steps:
[0053] Step 1: Obtain the EEG signals when humans show different emotions and perform low-pass filtering processing. The specific process is:
[0054] (1) Record the EEG signals and peripheral physiological signals of 32 subjects after watching 40 1-minute short music videos. The dataset is divided into 9 labels: Depressed, Calm, Relaxed, Sad, Peaceful, Joyful, Distressed, Excited, Satisfied. The dimension of each piece of data is 40×40×8064, the first 40 represents 40 different music videos watched by each subject, the second 40 represents the number of EEG channels, and 8064 is the recorded EEG signal data Points, each piece of data has a corresponding emotional label, represented by Arabic numerals 0-8.
[0055] (2) A low-pass filter is used to perform low-pass filtering of 0-30 Hz on the EEG signal to remove noise interference in the high-frequency band.
[0056] Step 2: Input the EEG signal...
PUM
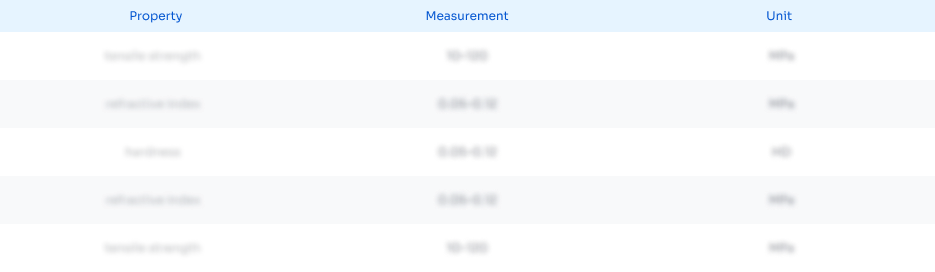
Abstract
Description
Claims
Application Information
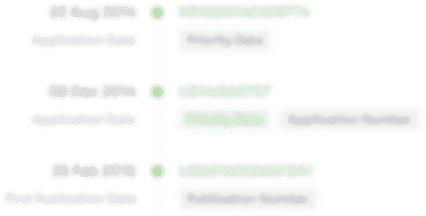
- R&D Engineer
- R&D Manager
- IP Professional
- Industry Leading Data Capabilities
- Powerful AI technology
- Patent DNA Extraction
Browse by: Latest US Patents, China's latest patents, Technical Efficacy Thesaurus, Application Domain, Technology Topic, Popular Technical Reports.
© 2024 PatSnap. All rights reserved.Legal|Privacy policy|Modern Slavery Act Transparency Statement|Sitemap|About US| Contact US: help@patsnap.com