Image super-resolution reconstruction method based on multi-band deep convolutional neural network
A deep convolution, neural network technology, applied in the image field, can solve the problems of gradient disappearance, high training difficulty, low flexibility, etc., to achieve the effect of enhancing image details, good reconstruction results, and fewer convolution layers
- Summary
- Abstract
- Description
- Claims
- Application Information
AI Technical Summary
Problems solved by technology
Method used
Image
Examples
Embodiment Construction
[0039] In order to make the purpose, technical solutions and advantages of the embodiments of the present invention clearer, the present invention will be described more completely below in conjunction with the accompanying drawings and specific embodiments. Herein, the schematic embodiments and descriptions of the present invention are used to explain the present invention, but not as a limitation to the present invention. Based on the embodiments of the present invention, those skilled in the art obtained without creative work All other embodiments belong to the protection scope of the present invention.
[0040] figure 1 A network model of an image super-resolution reconstruction method based on a multi-band deep convolutional neural network provided by the present invention, figure 2 It is the network model training flowchart of the present invention, image 3 For the reconstruction flow chart of processing the low-resolution image to be tested in the present invention,...
PUM
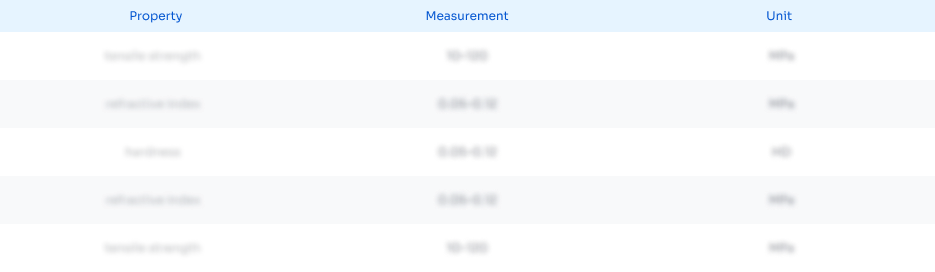
Abstract
Description
Claims
Application Information
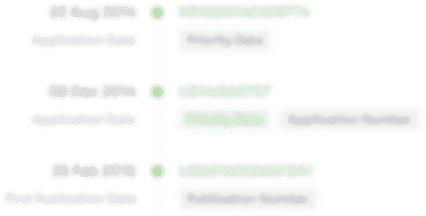
- R&D Engineer
- R&D Manager
- IP Professional
- Industry Leading Data Capabilities
- Powerful AI technology
- Patent DNA Extraction
Browse by: Latest US Patents, China's latest patents, Technical Efficacy Thesaurus, Application Domain, Technology Topic, Popular Technical Reports.
© 2024 PatSnap. All rights reserved.Legal|Privacy policy|Modern Slavery Act Transparency Statement|Sitemap|About US| Contact US: help@patsnap.com