Deep learning-based vehicle detection method
A technology of vehicle detection and deep learning, applied in the field of vehicle detection based on deep learning, can solve problems such as low efficiency and poor generalization ability
- Summary
- Abstract
- Description
- Claims
- Application Information
AI Technical Summary
Problems solved by technology
Method used
Image
Examples
Embodiment Construction
[0061] The present invention will be described in detail below with reference to the drawings and specific embodiments.
[0062] A vehicle detection method based on deep learning, such as figure 1 As shown, follow the steps below:
[0063] Step 1. Construct an image library with annotations and labels as the training sample set and the test sample set; specifically:
[0064] Use the open source calibration software LabelImg to construct an image library with labels and labels as the training sample set and test sample set, where the sample set contains vehicle photos (including background) taken under the same weather conditions in different scenarios and in different weather conditions. It is to record the coordinates of the upper left corner and the lower right corner of the vehicle in an entire image. The label refers to the category of the vehicle at each given vehicle position;
[0065] Step 2. Construct an improved Faster R-CNN model. The improved Faster R-CNN model consists of...
PUM
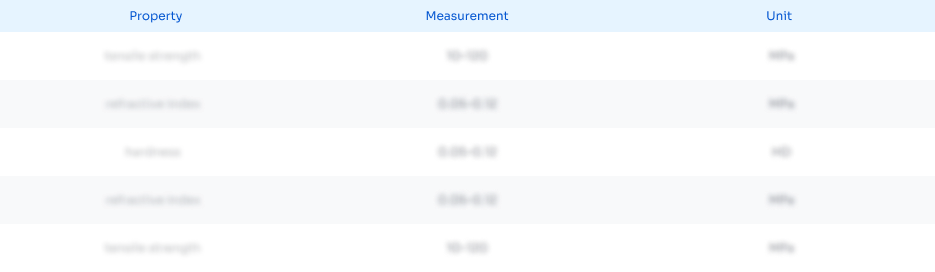
Abstract
Description
Claims
Application Information
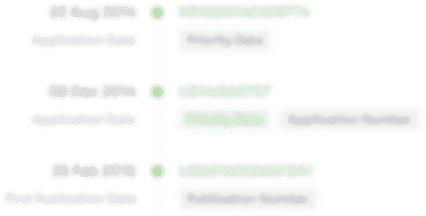
- R&D
- Intellectual Property
- Life Sciences
- Materials
- Tech Scout
- Unparalleled Data Quality
- Higher Quality Content
- 60% Fewer Hallucinations
Browse by: Latest US Patents, China's latest patents, Technical Efficacy Thesaurus, Application Domain, Technology Topic, Popular Technical Reports.
© 2025 PatSnap. All rights reserved.Legal|Privacy policy|Modern Slavery Act Transparency Statement|Sitemap|About US| Contact US: help@patsnap.com