Ensemble learning method based on self-adaptive sample expansion
A sample adaptive and integrated learning technology, applied in the field of remote sensing, can solve the problems of difficulty in obtaining real samples of remote sensing images, insufficient samples, etc., and achieve the effects of reducing manpower and time, solving under-learning, and good repeatability
- Summary
- Abstract
- Description
- Claims
- Application Information
AI Technical Summary
Problems solved by technology
Method used
Image
Examples
Embodiment Construction
[0042] The present invention will be further described in detail below in conjunction with the accompanying drawings, so that those skilled in the art can implement it with reference to the description.
[0043] It should be understood that terms such as "having", "comprising" and "including" used herein do not exclude the presence or addition of one or more other elements or combinations thereof.
[0044] The present invention is further illustrated below by specific examples. However, the specific details of the embodiments are only used to explain the present invention, and should not be construed as limiting the general technical solution of the present invention.
[0045] This embodiment provides an integrated learning method based on adaptive expansion of samples. figure 1 The main implementation methods of the present invention are illustrated: ① Combining the remote sensing image of the research area and the ground survey points to generate an image sample library, ca...
PUM
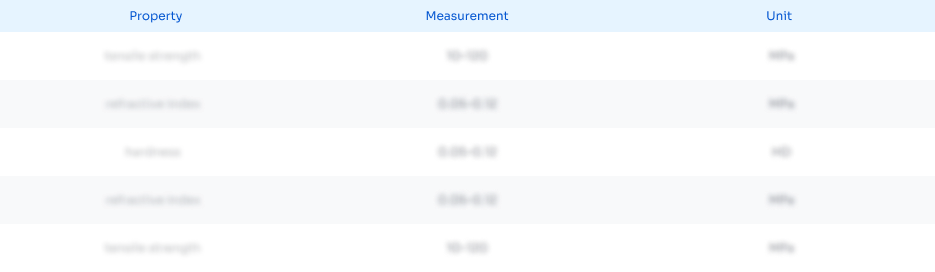
Abstract
Description
Claims
Application Information
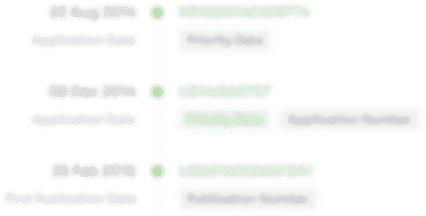
- Generate Ideas
- Intellectual Property
- Life Sciences
- Materials
- Tech Scout
- Unparalleled Data Quality
- Higher Quality Content
- 60% Fewer Hallucinations
Browse by: Latest US Patents, China's latest patents, Technical Efficacy Thesaurus, Application Domain, Technology Topic, Popular Technical Reports.
© 2025 PatSnap. All rights reserved.Legal|Privacy policy|Modern Slavery Act Transparency Statement|Sitemap|About US| Contact US: help@patsnap.com