Real image blind denoising method based on deep residual network
A real image and image technology, applied in image enhancement, image analysis, image data processing, etc., can solve the problems of nonlinear feature representation and image reconstruction, poor image denoising effect, and difficult model convergence.
- Summary
- Abstract
- Description
- Claims
- Application Information
AI Technical Summary
Problems solved by technology
Method used
Image
Examples
Embodiment Construction
[0099] In order to facilitate those of ordinary skill in the art to understand and implement the present invention, the present invention will be described in further detail below in conjunction with the accompanying drawings and embodiments. It should be understood that the implementation examples described here are only used to illustrate and explain the present invention, and are not intended to limit this invention.
[0100] The real image blind denoising method based on deep residual network in this embodiment, the specific process is as follows figure 1 shown, including the following steps:
[0101] Step 1: Select the clear image set in RGB space through the image data set, obtain the noisy image set in RGB space through spatial transformation, and construct the image set in RGB space through the clear image set in RGB space and the noisy image set in RGB space;
[0102] As preferably, described in step 1 selects and selects K=500 images in the image data set BSD (The Ber...
PUM
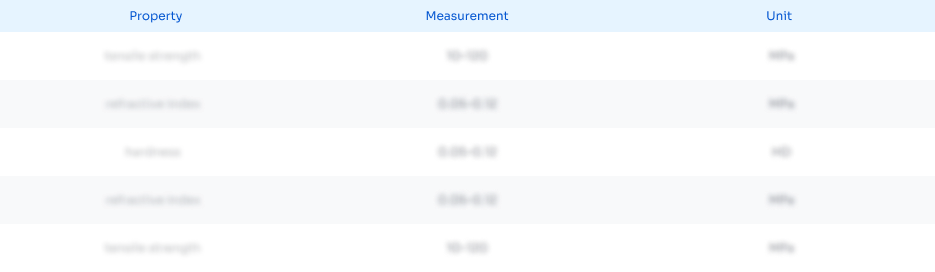
Abstract
Description
Claims
Application Information
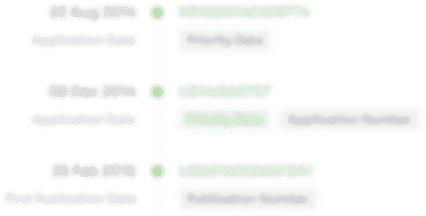
- R&D Engineer
- R&D Manager
- IP Professional
- Industry Leading Data Capabilities
- Powerful AI technology
- Patent DNA Extraction
Browse by: Latest US Patents, China's latest patents, Technical Efficacy Thesaurus, Application Domain, Technology Topic, Popular Technical Reports.
© 2024 PatSnap. All rights reserved.Legal|Privacy policy|Modern Slavery Act Transparency Statement|Sitemap|About US| Contact US: help@patsnap.com