Intelligent fault diagnosis method based on circulation neural network
A cyclic neural network and fault diagnosis technology, applied in the field of intelligent fault diagnosis based on cyclic neural network, can solve problems such as difficulty in maintaining accuracy, one-sided diagnosis results, and large manual workload, so as to enhance generalization ability and prevent overfitting. The effect of integration and high recognition speed
- Summary
- Abstract
- Description
- Claims
- Application Information
AI Technical Summary
Problems solved by technology
Method used
Image
Examples
Embodiment Construction
[0031] The present invention will be further described below in conjunction with the accompanying drawings.
[0032] figure 1 It is a flowchart of the intelligent fault diagnosis method based on the cyclic neural network of the present invention, such as figure 1 As shown, the method includes the following steps:
[0033] (1) Use the acceleration sensor to obtain the time-series vibration signals of the rotating machinery working in different health states: the vibration signal data is obtained through the engine-rotor test bench, and the test works in the form of motor-gearbox-load. The gear box is a one-stage planetary gear box, including 3 planetary gears, 1 sun gear and 1 fixed ring gear. The acceleration sensor is used to collect the vibration acceleration in the vertical direction in the test as the test processing signal. The test mainly collects the vibration signals of the planetary gear in four states: normal, tooth surface wear, broken tooth, and combined failure...
PUM
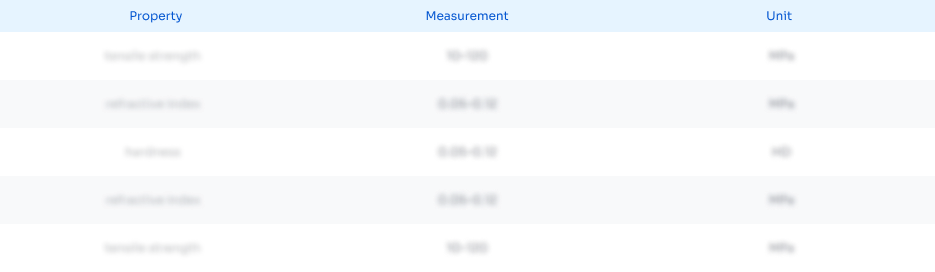
Abstract
Description
Claims
Application Information
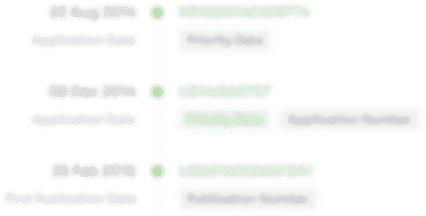
- Generate Ideas
- Intellectual Property
- Life Sciences
- Materials
- Tech Scout
- Unparalleled Data Quality
- Higher Quality Content
- 60% Fewer Hallucinations
Browse by: Latest US Patents, China's latest patents, Technical Efficacy Thesaurus, Application Domain, Technology Topic, Popular Technical Reports.
© 2025 PatSnap. All rights reserved.Legal|Privacy policy|Modern Slavery Act Transparency Statement|Sitemap|About US| Contact US: help@patsnap.com