A kind of cell detection method
A detection method and cell technology, applied in the field of cell detection, can solve the problems of large fluctuations in loss curves, slow calculation speed, and low accuracy of results, and achieve detection speed and calculation resource consumption, good detection accuracy and detection speed, The effect of small fluctuations in the loss curve
- Summary
- Abstract
- Description
- Claims
- Application Information
AI Technical Summary
Problems solved by technology
Method used
Image
Examples
Embodiment 1
[0093] In this embodiment, the original cell picture is cut into 2048*2048, and the specific cell is calibrated by an expert, and the specific type and cell location are marked, stored as an xml file, and the calibrated specific cell picture set is used as a training set for the picture Take training samples;
[0094] 2. Perform statistics and analysis on the area, aspect ratio and the number of specific cells in each specific cell in the training sample, and select the most suitable network parameters, which mainly include the anchor_size parameter and the anchor_scalse parameter;
[0095] 3. Set the network parameters and use the training samples to train the algorithm. Before entering the algorithm, the pictures are uniformly scaled to 600*800, and the training model and training parameters are saved, including loss parameters, accurate parameters, etc.;
[0096] 4. Put the picture of the cell to be detected (cut to 2048*2048) into the trained model for testing, and finally...
Embodiment 2
[0098] In this embodiment, we choose the resolution of the pictures to be 2048*2048, manually mark 98 specific cells in a total of 73 pictures, and select 58 pictures (there are 78 manually marked specific cells in 58 pictures) to pair The neural network is trained and tested with the remaining 15 pictures (20 of the 15 pictures have specific cells manually labeled).
[0099] In this embodiment, the YOLO network, the FASTER network and the multi-task-driven deep detection anchor network provided by the present invention are selected for training and testing. Finally, the loss curves of the three networks are obtained as Figure 4 shown.
[0100] It can be seen from the figure that the YOLO loss curve fluctuates greatly, and even after 1000 iterations, it still has a high loss. The multi-task-driven deep detection anchor network provided by the present invention is obviously better than the YOLO network.
[0101] It can be seen from the figure that the loss curve of the prese...
PUM
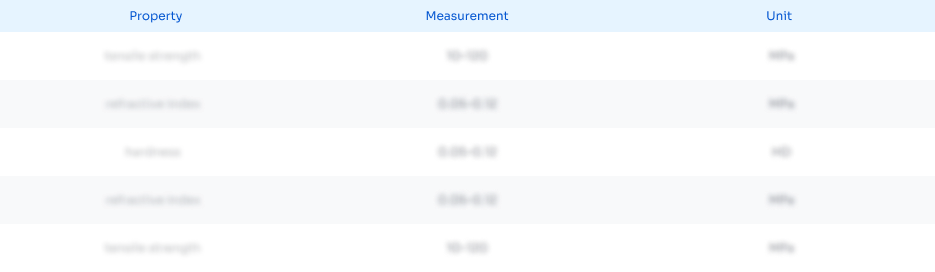
Abstract
Description
Claims
Application Information
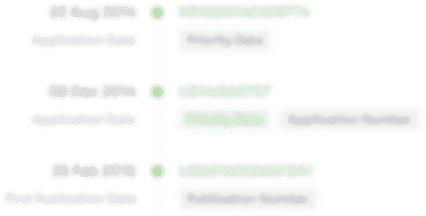
- R&D
- Intellectual Property
- Life Sciences
- Materials
- Tech Scout
- Unparalleled Data Quality
- Higher Quality Content
- 60% Fewer Hallucinations
Browse by: Latest US Patents, China's latest patents, Technical Efficacy Thesaurus, Application Domain, Technology Topic, Popular Technical Reports.
© 2025 PatSnap. All rights reserved.Legal|Privacy policy|Modern Slavery Act Transparency Statement|Sitemap|About US| Contact US: help@patsnap.com