A Neural Network Based Combined Forecasting Method for Bus Passenger Flow by Period
A combined forecasting and neural network technology, applied in neural learning methods, biological neural network models, forecasting, etc., to achieve the effect of improving overall forecasting performance, good generalization, and improved performance
- Summary
- Abstract
- Description
- Claims
- Application Information
AI Technical Summary
Problems solved by technology
Method used
Image
Examples
Embodiment approach
[0039] The first step is to collect basic relevant information. By analyzing the sample data, it is found that the bus passenger flow has three distribution characteristics: time, space and crowd. Combined with relevant literature, the present invention specifically collects the following information: The relevant information is divided into two categories. , objective influencing factors and relevant historical data.
[0040] Objective influencing factors include month M, Sunday change We, daily time change D, flat and peak peaks P, holidays H, and minimum temperature T 1 , the maximum temperature T2, the average temperature T, the wind direction Wi, the rainfall R; the relevant historical data includes the passenger flow Q in the two periods adjacent to the forecast period 11 , Q 12 , the passenger flow Q of the adjacent 3 working days 21 , Q 22 , Q 23 , the passenger flow Q of the same Sunday and the same time period in the adjacent 3 weeks 31 , Q 32 , Q 33 , the cor...
PUM
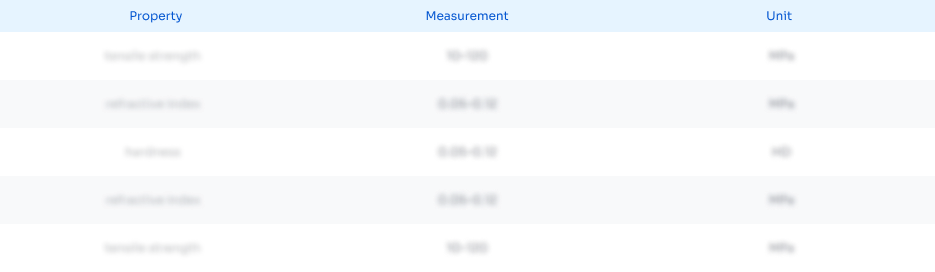
Abstract
Description
Claims
Application Information
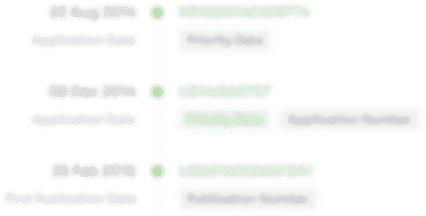
- R&D
- Intellectual Property
- Life Sciences
- Materials
- Tech Scout
- Unparalleled Data Quality
- Higher Quality Content
- 60% Fewer Hallucinations
Browse by: Latest US Patents, China's latest patents, Technical Efficacy Thesaurus, Application Domain, Technology Topic, Popular Technical Reports.
© 2025 PatSnap. All rights reserved.Legal|Privacy policy|Modern Slavery Act Transparency Statement|Sitemap|About US| Contact US: help@patsnap.com