Fine-grained zero-sample classification method based on multi-layer semantic supervised attention model
A technology of attention model and classification method, which is applied in the field of fine-grained zero-sample classification based on multi-layer semantic supervision attention model, and can solve problems such as limiting the scalability of classification models
- Summary
- Abstract
- Description
- Claims
- Application Information
AI Technical Summary
Problems solved by technology
Method used
Image
Examples
Embodiment Construction
[0036] The fine-grained zero-shot classification method based on the multi-layer semantic supervised attention model of the present invention will be described in detail below with reference to the embodiments and the accompanying drawings.
[0037] The fine-grained zero-sample classification method based on the multi-layer semantic supervised attention model of the present invention first uses the convolutional neural network to extract the local visual features of the selected parts in the fine-grained image, and uses the text description information of the category as the category The semantic feature supervises the classification of the local visual features of the fine-grained image, gradually assigns weights to the local visual features of the fine-grained image, and obtains a semantic supervision attention model, wherein the local visual features with higher correlation with semantic information, The corresponding weight is larger; use category semantic features to guide...
PUM
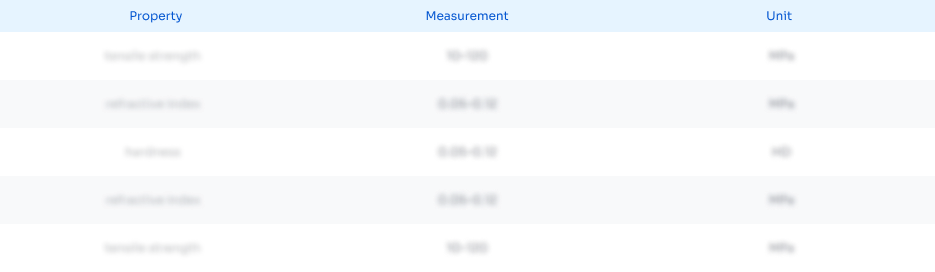
Abstract
Description
Claims
Application Information
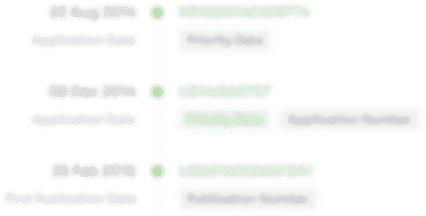
- R&D Engineer
- R&D Manager
- IP Professional
- Industry Leading Data Capabilities
- Powerful AI technology
- Patent DNA Extraction
Browse by: Latest US Patents, China's latest patents, Technical Efficacy Thesaurus, Application Domain, Technology Topic.
© 2024 PatSnap. All rights reserved.Legal|Privacy policy|Modern Slavery Act Transparency Statement|Sitemap