Automatic segmentation method based on PCANet depth learning model
A deep learning and automatic segmentation technology, which is applied in image analysis, character and pattern recognition, image enhancement, etc., can solve the problems that clustering methods cannot achieve segmentation accuracy, are not robust, and rely on grayscale information, etc.
- Summary
- Abstract
- Description
- Claims
- Application Information
AI Technical Summary
Problems solved by technology
Method used
Image
Examples
Embodiment 1
[0112] The present embodiment provides a kind of automatic segmentation technique of mammography mass under PCANet deep learning model, comprises the following steps, as figure 1 Shown:
[0113] Step 1: Input the test image into the first-level coarse segmentation network of the trained network model, obtain a set of label values of the image classification of the superpixel block of the test image, and fill back the corresponding position with the label value to obtain the binary value The pre-segmented template map;
[0114] Step 1-1 calculates the preprocessed image I used in the rough segmentation network 1 , according to formulas (1) and (2), the size of the structural elements in the top-hat transformation is about 35 pixels to obtain the image after reducing the background interference of breast tissue;
[0115] Step 1-2 calculation is used to obtain super pixel blocks by simple linear iterative clustering method inside the coarse segmentation network According to...
PUM
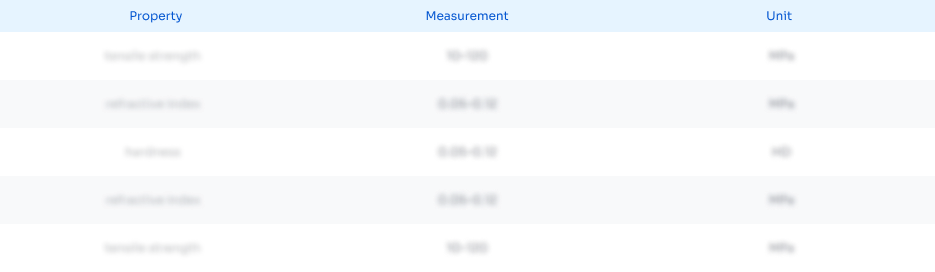
Abstract
Description
Claims
Application Information
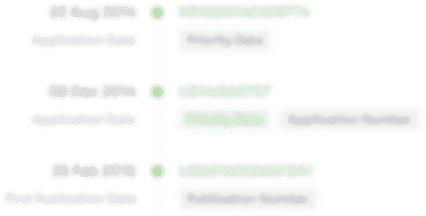
- R&D Engineer
- R&D Manager
- IP Professional
- Industry Leading Data Capabilities
- Powerful AI technology
- Patent DNA Extraction
Browse by: Latest US Patents, China's latest patents, Technical Efficacy Thesaurus, Application Domain, Technology Topic.
© 2024 PatSnap. All rights reserved.Legal|Privacy policy|Modern Slavery Act Transparency Statement|Sitemap