Cross-domain text sentiment classification method based on domain confrontation self-adaption
A sentiment classification and cross-domain technology, applied in the field of text analysis, can solve the problems of inability to accurately predict the emotional tendency of new comment data and low efficiency
- Summary
- Abstract
- Description
- Claims
- Application Information
AI Technical Summary
Problems solved by technology
Method used
Image
Examples
Embodiment Construction
[0020] The present invention will be described in further detail below in conjunction with specific embodiments and with reference to the accompanying drawings.
[0021] The method model structure of the present invention is as figure 1 As shown, the flow chart of the method is as figure 2 As shown, it specifically includes the following steps:
[0022] Step 1, input the word vector matrix, sentiment category label and domain label of the source domain and target domain samples.
[0023] Since the computer cannot directly process text data, it is necessary to convert the text input data into a data type recognizable by the computer. Let the number of rows n of the matrix represent the total number of words in the paragraph, and the number of columns of the matrix k represent the dimension of the word vector. First, convert each word in the input text into a 1×k word vector, and then follow the order in which the words appear in the text , concatenate the word vectors into ...
PUM
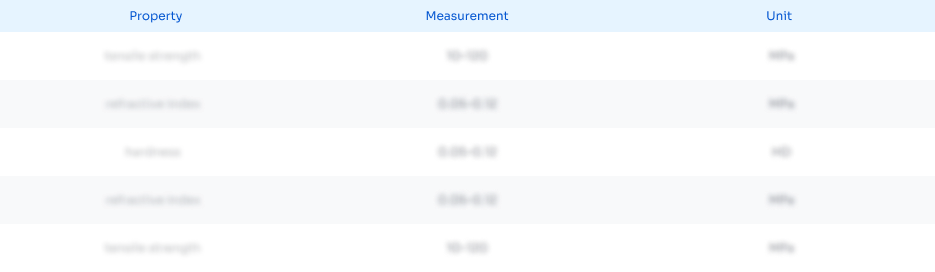
Abstract
Description
Claims
Application Information
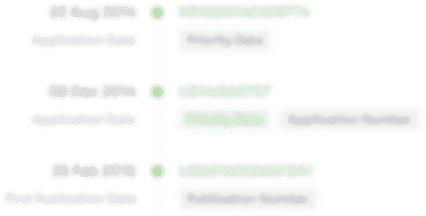
- R&D Engineer
- R&D Manager
- IP Professional
- Industry Leading Data Capabilities
- Powerful AI technology
- Patent DNA Extraction
Browse by: Latest US Patents, China's latest patents, Technical Efficacy Thesaurus, Application Domain, Technology Topic, Popular Technical Reports.
© 2024 PatSnap. All rights reserved.Legal|Privacy policy|Modern Slavery Act Transparency Statement|Sitemap|About US| Contact US: help@patsnap.com