A method for estimating air particulate pollution degree based on shallow convolution neural network
A convolutional neural network and air particulate matter technology, applied in neural learning methods, biological neural network models, neural architectures, etc., can solve problems such as high algorithm complexity, low detection accuracy, and slow training speed, so as to improve estimation accuracy, Improve training speed and prevent overfitting effect
- Summary
- Abstract
- Description
- Claims
- Application Information
AI Technical Summary
Problems solved by technology
Method used
Image
Examples
Embodiment Construction
[0054] In this scheme, a shallow convolutional neural network is selected to process the image. The specific idea is: the shallow convolutional neural network can extract local features such as texture and shape of the image, avoiding complicated steps such as feature extraction and feature optimization; Select part of the test set images to generate the corresponding heat map (Heatmap), such as Figure 4 As shown in the third column, by comparing with the original image, it can be found that the focus of image feature extraction is whether the image edge and texture are clear; the existing deep convolutional neural network model is suitable for object recognition tasks, while the shallow convolutional neural network is It can extract local features such as texture and shape of the image, so it is proposed to construct a shallow convolutional neural network model to estimate the PM2.5 index value of the image.
[0055] Such as Figure 1 to Figure 8 As shown, the present inven...
PUM
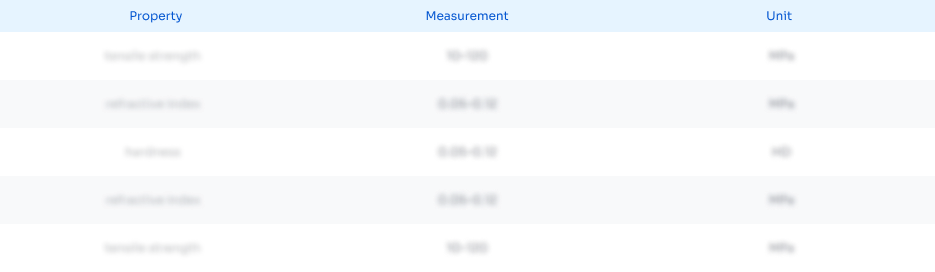
Abstract
Description
Claims
Application Information
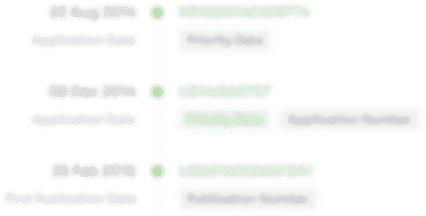
- R&D Engineer
- R&D Manager
- IP Professional
- Industry Leading Data Capabilities
- Powerful AI technology
- Patent DNA Extraction
Browse by: Latest US Patents, China's latest patents, Technical Efficacy Thesaurus, Application Domain, Technology Topic, Popular Technical Reports.
© 2024 PatSnap. All rights reserved.Legal|Privacy policy|Modern Slavery Act Transparency Statement|Sitemap|About US| Contact US: help@patsnap.com