Electrocardiosignal classifying method and system based on LRF-ELM and BLSTM
A LRF-ELM, ECG signal technology, used in medical science, sensors, diagnostic recording/measurement, etc., can solve the problems of deep learning algorithm memory consumption, affecting the classification effect, large amount of calculation, etc., to prevent insufficient computer memory , Good classification and recognition performance, efficient and fast extraction effect
- Summary
- Abstract
- Description
- Claims
- Application Information
AI Technical Summary
Problems solved by technology
Method used
Image
Examples
Embodiment 1
[0053] like Figure 1-6 As shown, Embodiment 1 of the present disclosure provides an ECG signal classification method based on LRF-ELM and BLSTM, the steps are as follows,
[0054] Obtain and preprocess the ECG signal data to obtain a data set, and use the ECG signal data in the data set as the input data of the neural network;
[0055] Using the LRF-ELM network as a feature extractor, it learns the spatial information in the ECG signal data, and extracts the feature data of different dimensions in the ECG signal data through three stacked random convolution and pooling processes;
[0056] The extracted feature data is fused as the input of the sequence learning stage, and the deep BLSTM network is used for sequence learning, and finally the ECG signal classification result is output.
[0057] In this embodiment, its performance is verified on the MIT-BIH data set. In this embodiment, 99863 samples in the data set are first divided, and each type of sample in the data set is ...
Embodiment 2
[0092] Embodiment 2 of the present disclosure provides an ECG signal classification system based on LRF-ELM and BLSTM, using the ECG signal classification method based on LRF-ELM and BLSTM described in Embodiment 1, including:
[0093] The preprocessing module is configured to: obtain and preprocess the ECG signal data to obtain a data set, and use the ECG signal data in the data set as the input data of the neural network;
[0094] The feature extraction module is configured to: use the LRF-ELM network as a feature extractor to learn the spatial information in the ECG signal data, and extract different dimensions of the ECG signal data through three stacked random convolution and pooling processes feature;
[0095] The sequence learning module is configured to: use the extracted features as the input of the sequence learning stage after fusion, use the deep BLSTM network for sequence learning, and finally output the ECG signal classification result.
Embodiment 3
[0097] Embodiment 3 of the present disclosure provides a medium on which a program is stored. When the program is executed by a processor, the steps in the ECG signal classification method based on LRF-ELM and BLSTM as described in Embodiment 1 of the present disclosure are implemented.
PUM
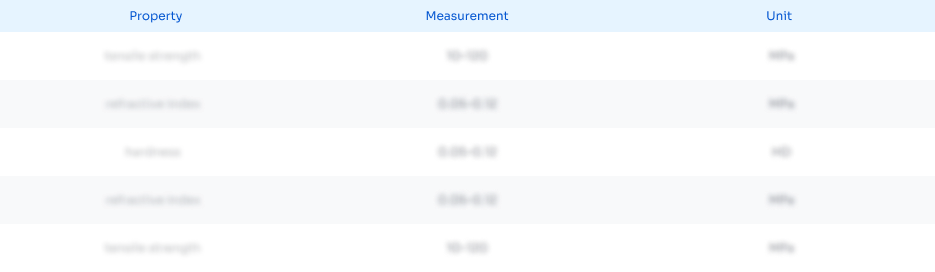
Abstract
Description
Claims
Application Information
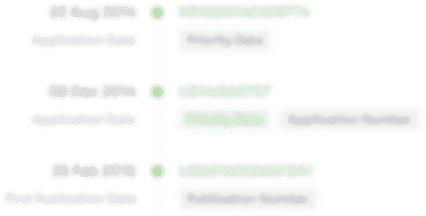
- R&D
- Intellectual Property
- Life Sciences
- Materials
- Tech Scout
- Unparalleled Data Quality
- Higher Quality Content
- 60% Fewer Hallucinations
Browse by: Latest US Patents, China's latest patents, Technical Efficacy Thesaurus, Application Domain, Technology Topic, Popular Technical Reports.
© 2025 PatSnap. All rights reserved.Legal|Privacy policy|Modern Slavery Act Transparency Statement|Sitemap|About US| Contact US: help@patsnap.com