A compression perceptual image reconstruction method based on Generative Adversarial Networks based on generation antagonism network
A technology of compressed sensing and image reconstruction, applied in biological neural network models, image data processing, neural learning methods, etc., can solve problems such as long reconstruction time and poor quality of reconstructed images, and achieve the effect of accurately reconstructing original images
- Summary
- Abstract
- Description
- Claims
- Application Information
AI Technical Summary
Problems solved by technology
Method used
Image
Examples
Embodiment Construction
[0049] Such as figure 1 As shown, the present invention provides a compressive sensing image reconstruction method based on generative confrontation network, including:
[0050] Step 1. Sampling the original image to obtain the measurement vector
[0051] Measurement vector y=Φx+ξ, y∈R M , Φ∈R M×N , x∈R N , y represents the measurement vector, Φ is the measurement vector, x represents the data row vector after the image data matrix becomes vectorized, M represents the size of the measurement vector, and N is the number of pixels in the original image.
[0052]Step 2. Construct a generative confrontation network model according to the size of the measurement vector and the size of the reconstructed image, and design an objective function for optimizing network model parameters.
[0053] Among them, the size of the measurement vector determines the size of the input of the generator, and the size of the reconstructed image determines the final output size of the generator. ...
PUM
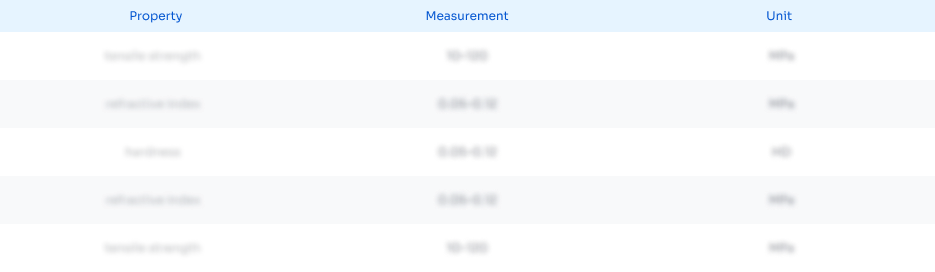
Abstract
Description
Claims
Application Information
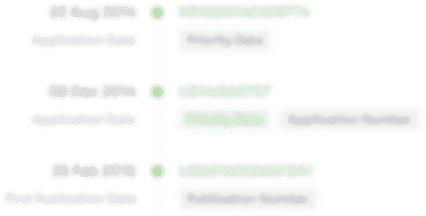
- R&D
- Intellectual Property
- Life Sciences
- Materials
- Tech Scout
- Unparalleled Data Quality
- Higher Quality Content
- 60% Fewer Hallucinations
Browse by: Latest US Patents, China's latest patents, Technical Efficacy Thesaurus, Application Domain, Technology Topic, Popular Technical Reports.
© 2025 PatSnap. All rights reserved.Legal|Privacy policy|Modern Slavery Act Transparency Statement|Sitemap|About US| Contact US: help@patsnap.com