Long-term drift error compensation method for gravity sensor
A technology of drift error and compensation method, which is applied in the direction of instruments, scientific instruments, and sound wave reradiation, etc., and can solve the problems that the model cannot include data features and the recognition accuracy is not high
- Summary
- Abstract
- Description
- Claims
- Application Information
AI Technical Summary
Problems solved by technology
Method used
Image
Examples
Embodiment Construction
[0060] The technical solutions of the present invention will be further described below in conjunction with the drawings and embodiments.
[0061] The invention relates to a long-term random drift error compensation method of a gravity sensor, real-time quantitative prediction, radial symmetric scalar tree network modeling, mean value clustering analysis and drift error compensation method. This method overcomes the problem of poor identification and modeling of nonlinear systems in traditional real-time quantitative prediction modeling through radial symmetric scalar tree network modeling and mean value clustering analysis, and improves the radial symmetric scalar tree by combining real-time quantitative prediction model parameters. The recognition accuracy and robustness of the network.
[0062] Such as figure 1 As shown, a long-term drift error compensation method for a gravity sensor includes the following steps:
[0063] (1) Use the gravity data sampling samples of the ...
PUM
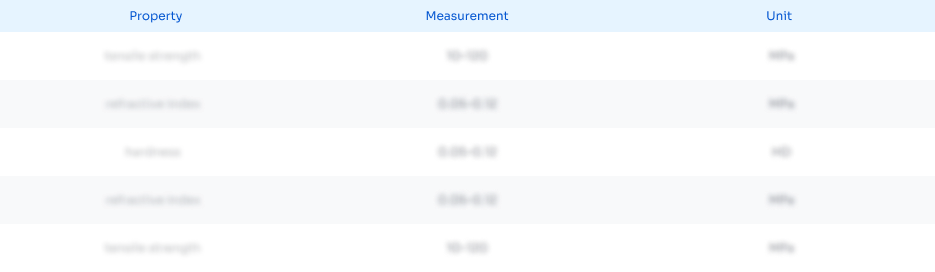
Abstract
Description
Claims
Application Information
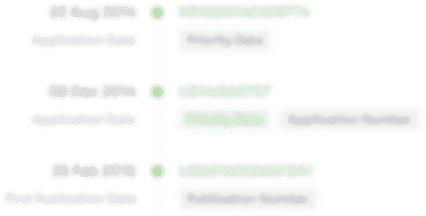
- R&D
- Intellectual Property
- Life Sciences
- Materials
- Tech Scout
- Unparalleled Data Quality
- Higher Quality Content
- 60% Fewer Hallucinations
Browse by: Latest US Patents, China's latest patents, Technical Efficacy Thesaurus, Application Domain, Technology Topic, Popular Technical Reports.
© 2025 PatSnap. All rights reserved.Legal|Privacy policy|Modern Slavery Act Transparency Statement|Sitemap|About US| Contact US: help@patsnap.com