Semi-supervised learning cell segmentation method based on a generative adversarial network
A semi-supervised learning, cell technology, applied in the field of cell segmentation of semi-supervised learning, can solve problems such as dependencies
- Summary
- Abstract
- Description
- Claims
- Application Information
AI Technical Summary
Problems solved by technology
Method used
Image
Examples
Embodiment Construction
[0063] The embodiments of the present invention are described in detail below. This embodiment is implemented on the premise of the technical solution of the present invention, and detailed implementation methods and specific operating procedures are provided, but the protection scope of the present invention is not limited to the following implementation example.
[0064] This embodiment discloses a cell segmentation method based on semi-supervised learning of confrontation generation network, comprising the following steps:
[0065] Step 1. Collect cell images, and divide the cell images into two parts, a training set and a verification set, wherein, some of the cell images in the training set are pre-labeled cell images, and the remaining cell images in the training set are non- Annotated cell images; the cell images in the verification set are all pre-labeled verification cell images; the cell image is specifically marked as a binary mask image.
[0066] Preferably: perfo...
PUM
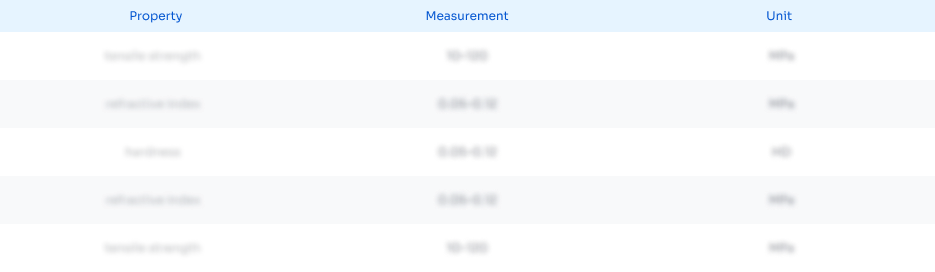
Abstract
Description
Claims
Application Information
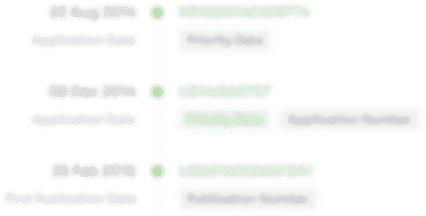
- R&D Engineer
- R&D Manager
- IP Professional
- Industry Leading Data Capabilities
- Powerful AI technology
- Patent DNA Extraction
Browse by: Latest US Patents, China's latest patents, Technical Efficacy Thesaurus, Application Domain, Technology Topic, Popular Technical Reports.
© 2024 PatSnap. All rights reserved.Legal|Privacy policy|Modern Slavery Act Transparency Statement|Sitemap|About US| Contact US: help@patsnap.com