Hyperspectral image super-resolution processing method based on a convolutional network
A hyperspectral image and convolutional network technology, applied in the field of image super-resolution processing, can solve problems that affect the resolution of high-resolution images, do not take into account the information of high-resolution/low-resolution image blocks, and do not take into account model uncertainty factors, etc. , to achieve the effect of increasing the resolution
- Summary
- Abstract
- Description
- Claims
- Application Information
AI Technical Summary
Problems solved by technology
Method used
Image
Examples
Embodiment Construction
[0027] The present invention will be further described below in conjunction with the accompanying drawings.
[0028] refer to figure 1 , The specific implementation steps of the present invention are as follows.
[0029] Step 1. Image acquisition, generate samples.
[0030] (1a) Obtain a low-resolution hyperspectral image L and a high-resolution multispectral image H from the Harvard image set;
[0031] (1b) The low-resolution hyperspectral image L and the high-resolution multispectral image H are used as training samples, and the low-resolution hyperspectral image L and high-resolution multispectral image H that are different from the training samples are used as test samples.
[0032] Step 2. Build a convolutional network.
[0033] (2a) Set up the inference sub-network, which is composed of the input as Hidden variable is A variational autoencoder network composed of two convolutional neural networks CNN, where Represents the i-th pixel of the low-resolution hypersp...
PUM
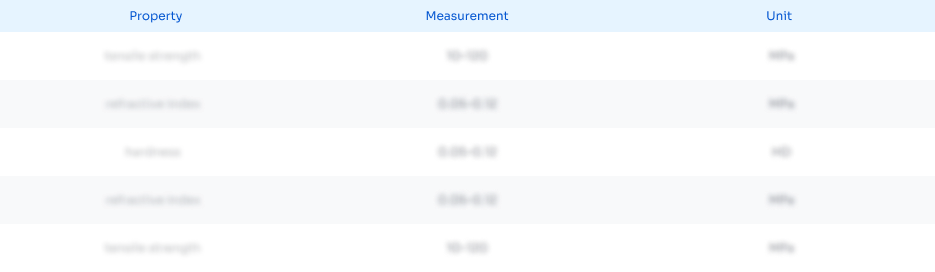
Abstract
Description
Claims
Application Information
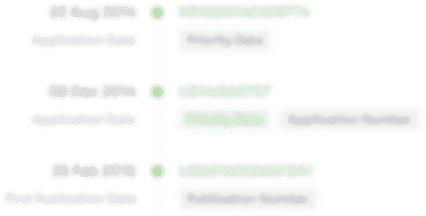
- R&D Engineer
- R&D Manager
- IP Professional
- Industry Leading Data Capabilities
- Powerful AI technology
- Patent DNA Extraction
Browse by: Latest US Patents, China's latest patents, Technical Efficacy Thesaurus, Application Domain, Technology Topic, Popular Technical Reports.
© 2024 PatSnap. All rights reserved.Legal|Privacy policy|Modern Slavery Act Transparency Statement|Sitemap|About US| Contact US: help@patsnap.com