An abnormal behavior detection method based on a linear dynamic system and a deep network
A dynamic system and detection method technology, applied in the field of video detection, can solve the problems of less nonlinear structure, lack of consideration of temporal relationship between time and space features, and low operation speed, so as to increase feature extraction capabilities, reduce network parameters, The effect of improving the fitting ability
- Summary
- Abstract
- Description
- Claims
- Application Information
AI Technical Summary
Problems solved by technology
Method used
Image
Examples
Embodiment 1
[0038] Example 1: Abnormal campus behavior detection: the overall process is as follows Figure 4 Shown:
[0039] Obtain video clips in key places such as campus corridors, classrooms, and cafeterias, such as fighting, running, climbing over guardrails, and talking. Filter the video samples and keep the samples with distinctive features as training samples. Classify the acquired videos according to the type of action, and mark the time points when the action occurs and ends. We believe that the video clips within the duration of the action can be used as positive samples, and the video clips that do not occur or do not contain detected actions can be used as negative samples. . The positive samples should have action videos of various appropriate proportions. Cut the obtained video samples into video segments of equal length, and perform normalization processing. Each segment contains m RGB images, and then calculate the corresponding optical flow video segment. The optical...
PUM
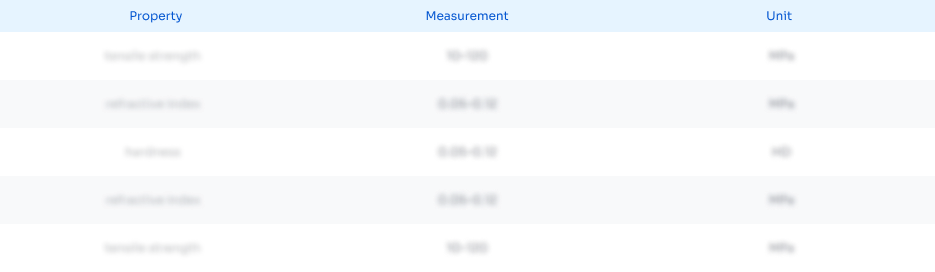
Abstract
Description
Claims
Application Information
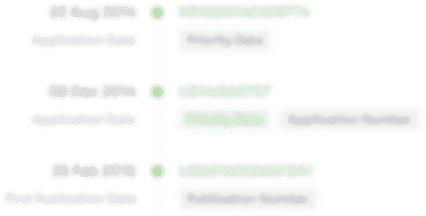
- R&D
- Intellectual Property
- Life Sciences
- Materials
- Tech Scout
- Unparalleled Data Quality
- Higher Quality Content
- 60% Fewer Hallucinations
Browse by: Latest US Patents, China's latest patents, Technical Efficacy Thesaurus, Application Domain, Technology Topic, Popular Technical Reports.
© 2025 PatSnap. All rights reserved.Legal|Privacy policy|Modern Slavery Act Transparency Statement|Sitemap|About US| Contact US: help@patsnap.com