An online comment fine-grained emotion analysis method based on multi-task learning
A multi-task learning and sentiment analysis technology, applied in the field of natural language processing, can solve the problems of inability to classify multiple fine-grained emotions at the same time, low accuracy, overfitting, etc., to reduce training time and prediction time, and reduce accuracy. , the effect of reducing the impact of noise
- Summary
- Abstract
- Description
- Claims
- Application Information
AI Technical Summary
Problems solved by technology
Method used
Image
Examples
Embodiment 1
[0075] 1. Introduction to the processing process:
[0076] Step 1, text segmentation and removal of stop words.
[0077] Chinese word segmentation refers to dividing Chinese sequences into individual words. Use the jieba tool for Chinese word segmentation. Stop words refer to words with no practical meaning such as "的" and "how" in Chinese, and other stop words can also be added manually. Delete these words from the data set according to the preset stop vocabulary.
[0078] Step 2, train word vectors, and map words to numbers.
[0079] Sentiment analysis technology based on deep learning needs to represent all words as low-dimensional dense vectors. Here, word2vec technology is used to train Chinese word vectors. Set the parameters of word2vec: the window size is set to 5, the minimum word frequency is set to 2, and the word vector dimension is set to 128. The word2vec technique is a model used to generate word vectors, wherein words whose occurrence times are lower than...
PUM
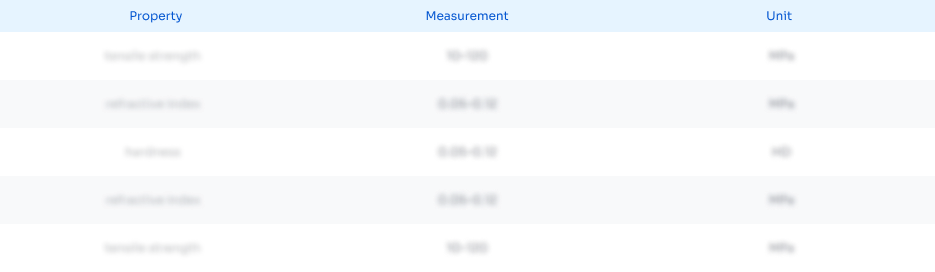
Abstract
Description
Claims
Application Information
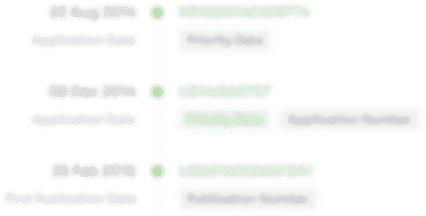
- R&D
- Intellectual Property
- Life Sciences
- Materials
- Tech Scout
- Unparalleled Data Quality
- Higher Quality Content
- 60% Fewer Hallucinations
Browse by: Latest US Patents, China's latest patents, Technical Efficacy Thesaurus, Application Domain, Technology Topic, Popular Technical Reports.
© 2025 PatSnap. All rights reserved.Legal|Privacy policy|Modern Slavery Act Transparency Statement|Sitemap|About US| Contact US: help@patsnap.com