Electric load forecasting method and system based on convolutional long-short-term memory neural network
A long-short-term memory and neural network technology, applied in the field of electric load forecasting based on convolutional long-short-term memory neural network, can solve the problems of low accuracy of electric load forecasting, sensitive data changes, and easy over-fitting, etc., to achieve Reduce the risk of model overfitting, improve robustness, and improve the effect of accuracy
- Summary
- Abstract
- Description
- Claims
- Application Information
AI Technical Summary
Problems solved by technology
Method used
Image
Examples
Embodiment 1
[0030] In the technical solutions disclosed in one or more embodiments, such as figure 1 As shown, the electricity load forecasting method based on the convolutional long short-term memory neural network includes the following steps:
[0031] Step 1. Obtain and preprocess the historical power consumption data of the power consumption system to be predicted;
[0032] Step 2. Transfer the preprocessed sample data to the trained multi-layer neural network including the MVCNN model and the ConvLSTM model, and output the prediction result;
[0033] Step 3. Output the power distribution control scheme according to the prediction result.
[0034] Optionally, the power distribution control scheme includes power distribution time allocation, power distribution area coordination, etc., and controls the control switches of corresponding lines according to the prediction results, so as to realize intelligent power distribution of each power distribution branch. Especially suitable for s...
Embodiment 2
[0102] This embodiment provides a power load forecasting system based on a convolutional long-short-term memory neural network, including:
[0103] Data acquisition and preprocessing module: configured to obtain historical power consumption data of the power consumption system to be predicted and perform preprocessing;
[0104] Prediction module: configured to transmit the preprocessed sample data to the trained multi-layer neural network including the MVCNN model and the ConvLSTM model, and output the prediction result;
[0105] Power distribution control output module: configured to output a power distribution control scheme according to a prediction result.
Embodiment 3
[0107] This embodiment provides an electronic device, including a memory, a processor, and computer instructions stored in the memory and executed on the processor. When the computer instructions are executed by the processor, the steps described in the method in Embodiment 1 are completed.
PUM
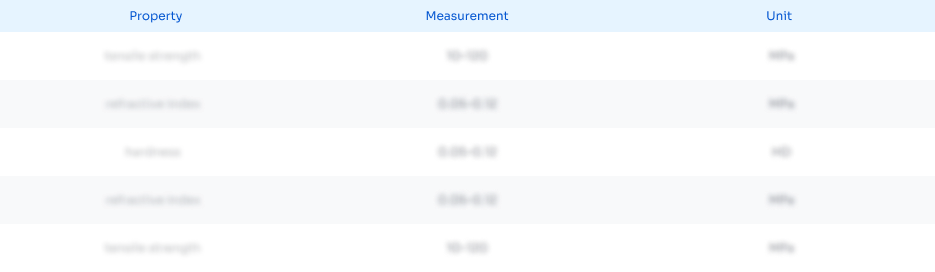
Abstract
Description
Claims
Application Information
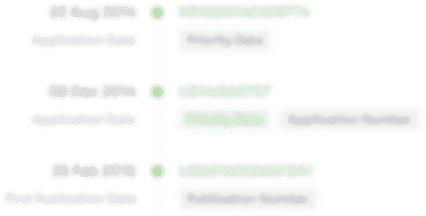
- R&D
- Intellectual Property
- Life Sciences
- Materials
- Tech Scout
- Unparalleled Data Quality
- Higher Quality Content
- 60% Fewer Hallucinations
Browse by: Latest US Patents, China's latest patents, Technical Efficacy Thesaurus, Application Domain, Technology Topic, Popular Technical Reports.
© 2025 PatSnap. All rights reserved.Legal|Privacy policy|Modern Slavery Act Transparency Statement|Sitemap|About US| Contact US: help@patsnap.com