Equipment fault diagnosis method based on multi-source signals and deep learning
A technology of deep learning and equipment failure, applied in neural learning methods, character and pattern recognition, instruments, etc., can solve problems such as insufficient robustness of single signal fault diagnosis, improve fitting ability and diagnostic performance, and improve training efficiency , Improving the effect of generalization ability and adaptability
- Summary
- Abstract
- Description
- Claims
- Application Information
AI Technical Summary
Problems solved by technology
Method used
Image
Examples
Embodiment Construction
[0028] The present invention will be described in detail below in conjunction with the accompanying drawings and specific embodiments. This embodiment is carried out on the premise of the technical solution of the present invention, and detailed implementation and specific operation process are given, but the protection scope of the present invention is not limited to the following embodiments.
[0029] For the convenience of the following description, let x=(x i,j,k ) H×H×W Represents a set of feature maps with N channels and a size of H×W, where H and W represent the number of rows and columns of a two-dimensional matrix, respectively. x i Denotes the feature map of the i-th channel, x i,j,k Represents the normalized element of row j and column k in the i-th channel, and has, i∈[1,N],j∈[1,H],k∈[1,W]. Note that since the number of convolution kernels in each layer is different, the number of feature map channels N of the input and output of each convolution layer changes....
PUM
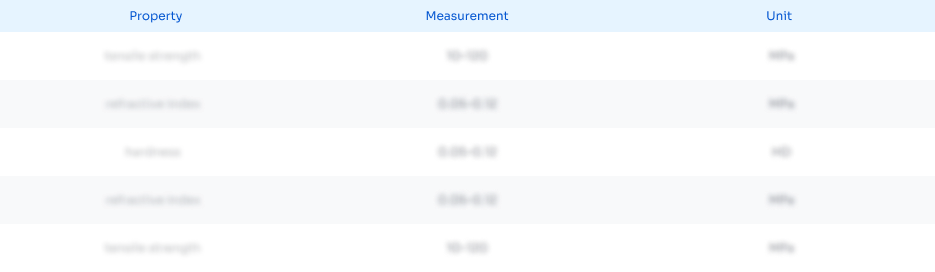
Abstract
Description
Claims
Application Information
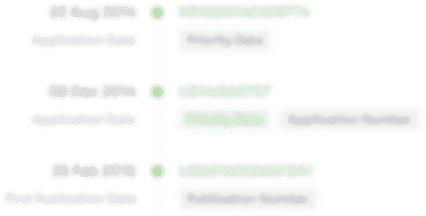
- R&D
- Intellectual Property
- Life Sciences
- Materials
- Tech Scout
- Unparalleled Data Quality
- Higher Quality Content
- 60% Fewer Hallucinations
Browse by: Latest US Patents, China's latest patents, Technical Efficacy Thesaurus, Application Domain, Technology Topic, Popular Technical Reports.
© 2025 PatSnap. All rights reserved.Legal|Privacy policy|Modern Slavery Act Transparency Statement|Sitemap|About US| Contact US: help@patsnap.com