Machine learning-based vehicle abnormal trajectory real-time recognition method
A recognition method and machine learning technology, which is applied in the field of abnormal vehicle trajectory recognition, can solve the problems of abnormal trajectory recognition models that cannot be updated and low recognition accuracy, and achieve real-time and efficient data processing, high detection efficiency, and improved recognition accuracy.
- Summary
- Abstract
- Description
- Claims
- Application Information
AI Technical Summary
Problems solved by technology
Method used
Image
Examples
Embodiment 1
[0066] Explanation of technical terms:
[0067] PMML, the full name of Predictive Model Markup Language (Predictive Model Markup Language), uses XML to describe and store data mining models. PMML is a de facto standard language for presenting data mining models.
[0068] Hadoop is a distributed system infrastructure developed by the Apache Foundation.
[0069] HDFS, Hadoop implements a distributed file system (Hadoop Distributed File System), referred to as HDFS. HDFS has the characteristics of high fault tolerance and is designed to be deployed on low-cost (low-cost) hardware; and it provides high throughput (high throughput) to access application data, suitable for those with large data sets (large data set) applications.
[0070] Hive, a Hadoop-based data warehouse tool, can map structured data files into a database table, and provides a simple SQL query function, which can convert SQL statements into MapReduce tasks for execution.
[0071] Spark, Apache Spark is a fast ...
Embodiment 2
[0108] On the basis of Embodiment 1 of the present invention, the refinement model is updated, such as Figure 4 As shown, the details are as follows:
[0109]Step 4. Model update:
[0110] Automatically updating and correcting the model according to the feedback information of the car owner includes the following steps:
[0111] Step aaa: based on the historical data and the latest data of the vehicle, automatically perform regular training on the outlier detection model to obtain a new model;
[0112] Step bbb: Automatically detect whether the accuracy of the new model meets the standard, if it meets the standard, skip to step ccc to update the model, if not, do not update the model;
[0113] Step ccc: Automatically replace and deploy the model that meets the detection standard.
[0114] In step aaa, the automatic regular training of the outlier detection model includes the following steps:
[0115] Build the training data set of model, described training data set compri...
PUM
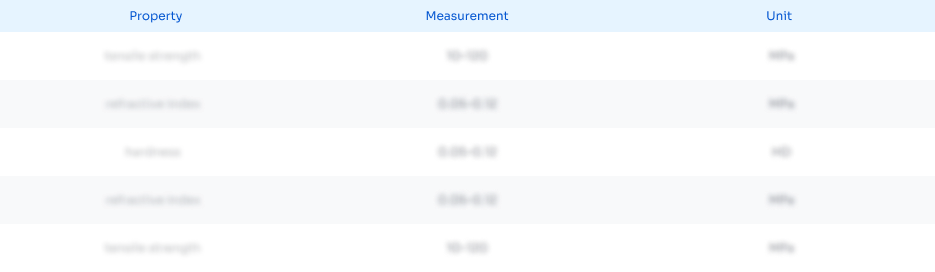
Abstract
Description
Claims
Application Information
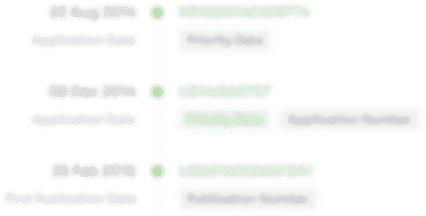
- R&D Engineer
- R&D Manager
- IP Professional
- Industry Leading Data Capabilities
- Powerful AI technology
- Patent DNA Extraction
Browse by: Latest US Patents, China's latest patents, Technical Efficacy Thesaurus, Application Domain, Technology Topic, Popular Technical Reports.
© 2024 PatSnap. All rights reserved.Legal|Privacy policy|Modern Slavery Act Transparency Statement|Sitemap|About US| Contact US: help@patsnap.com