small-area-level ultra-short-term load prediction and visualization method based on a deep LSTM network
A load forecasting and ultra-short-term technology, applied in forecasting, neural learning methods, biological neural network models, etc., can solve problems such as surges in operating costs
- Summary
- Abstract
- Description
- Claims
- Application Information
AI Technical Summary
Problems solved by technology
Method used
Image
Examples
Embodiment Construction
[0057] Embodiments of the present invention will be described in detail below with reference to the accompanying drawings.
[0058] A small area-level ultra-short-term load forecasting method based on deep LSTM, the flow chart of the method is as follows figure 1 As shown, the method includes the following steps:
[0059] Step 1: Determine the input and output variables of the model.
[0060] The determination of the input and output data sets is the key to determining the performance of the model. The ultra-short-term load forecasting in the power system is generally carried out in a point-by-point manner. The output of the model For the load value to be predicted, the interval of prediction points can be 15min, 30min or 1h. The input data refers to various attributes that affect the load. Before determining the input attributes, it is often necessary to know the approximate composition of the load and demand-side management information, such as whether to use pe...
PUM
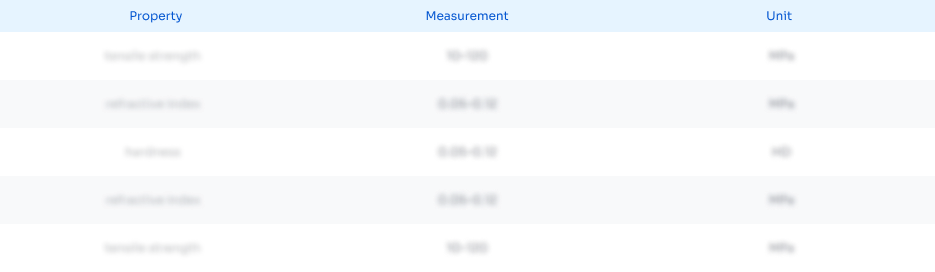
Abstract
Description
Claims
Application Information
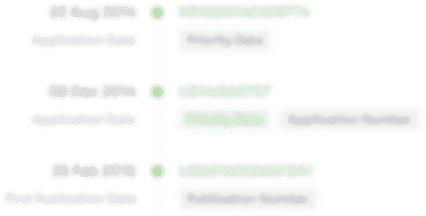
- R&D
- Intellectual Property
- Life Sciences
- Materials
- Tech Scout
- Unparalleled Data Quality
- Higher Quality Content
- 60% Fewer Hallucinations
Browse by: Latest US Patents, China's latest patents, Technical Efficacy Thesaurus, Application Domain, Technology Topic, Popular Technical Reports.
© 2025 PatSnap. All rights reserved.Legal|Privacy policy|Modern Slavery Act Transparency Statement|Sitemap|About US| Contact US: help@patsnap.com