A fan design second-generation algorithm multi-objective optimization method based on variable learning rate network modeling
A multi-objective optimization and network modeling technology, applied in the field of multi-objective optimization of the second-generation algorithm for wind turbine design, can solve problems such as difficult to achieve accurate and effective design, and low accuracy
- Summary
- Abstract
- Description
- Claims
- Application Information
AI Technical Summary
Problems solved by technology
Method used
Image
Examples
Embodiment Construction
[0074] The present invention will be further described below in conjunction with the accompanying drawings.
[0075] refer to figure 1 , a multi-objective optimization method of fan design second-generation algorithm for network modeling with variable learning rate, including the following steps:
[0076] Step 1: Collect the structural variables that have a great influence on the operating efficiency and cost of the fan, while the wind pressure and air volume are given values, and the efficiency and cost are the target variables. The data samples of the structural variables and target variables can be obtained through experiments;
[0077] Step 2: Let the structural variable be the input variable and the target variable be the output variable, train the data samples, and complete the establishment of the variable learning rate network model, wherein the variable learning rate method is used to update the weights and thresholds;
[0078] Step 3: Establish a second-generation a...
PUM
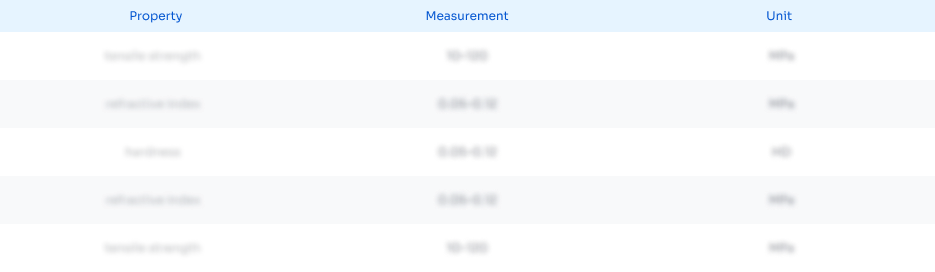
Abstract
Description
Claims
Application Information
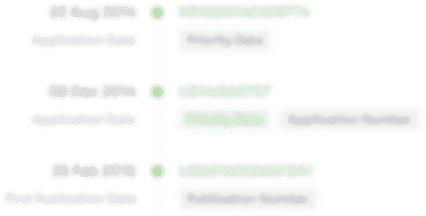
- R&D Engineer
- R&D Manager
- IP Professional
- Industry Leading Data Capabilities
- Powerful AI technology
- Patent DNA Extraction
Browse by: Latest US Patents, China's latest patents, Technical Efficacy Thesaurus, Application Domain, Technology Topic, Popular Technical Reports.
© 2024 PatSnap. All rights reserved.Legal|Privacy policy|Modern Slavery Act Transparency Statement|Sitemap|About US| Contact US: help@patsnap.com