Video emotion recognition method based on local enhanced motion history map and recursive convolutional neural network
A technology of motion history and local enhancement, applied in the field of pattern recognition, can solve the problems of low network classification ability, inability to make good use of video motion information, and small amount of data in facial expression video datasets, so as to prevent the small amount of training data, Improving the generalization ability and the effect of improving the classification ability
- Summary
- Abstract
- Description
- Claims
- Application Information
AI Technical Summary
Problems solved by technology
Method used
Image
Examples
Embodiment Construction
[0052] In this example, if figure 1 As shown, a video emotion recognition method based on local enhanced motion history graph and recursive convolutional neural network, including the following steps: obtain static expression picture data set and expression video data set, perform data expansion on video, and express expression video data set for preprocessing. A Local Enhanced Motion History Map (LEMHI) is then computed. Use the static image data set to pre-train the convolutional neural network (VGG16) model, the model structure is as follows figure 2 shown; then use LEMHI to fine-tune the pre-trained VGG16 model to obtain the LEMHI-CNN model. At the same time, the video frame is input into the pre-trained VGG16 model to extract spatial features, and the spatial features are stacked, sliced and pooled to train the CNN-LSTM neural network model. Finally, the weighted fusion of the recognition results of the LEMHI-CNN model and the CNN-LSTM model is used to obtain the fi...
PUM
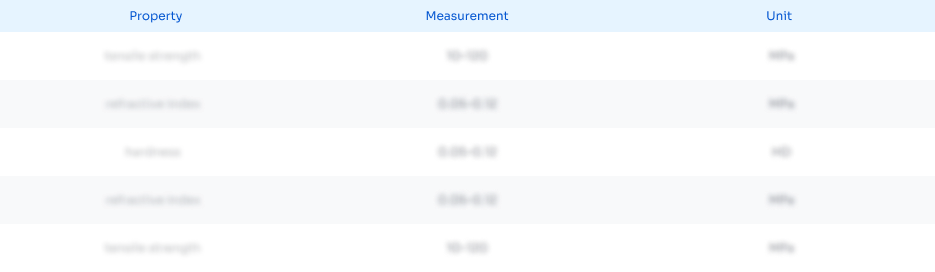
Abstract
Description
Claims
Application Information
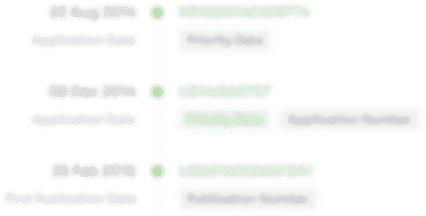
- R&D Engineer
- R&D Manager
- IP Professional
- Industry Leading Data Capabilities
- Powerful AI technology
- Patent DNA Extraction
Browse by: Latest US Patents, China's latest patents, Technical Efficacy Thesaurus, Application Domain, Technology Topic, Popular Technical Reports.
© 2024 PatSnap. All rights reserved.Legal|Privacy policy|Modern Slavery Act Transparency Statement|Sitemap|About US| Contact US: help@patsnap.com