A continuous action online learning control method and system for an autonomous vehicle
A technology of automatic driving and learning control, which is applied in the field of environmental perception of autonomous driving vehicles. It can solve problems such as insufficient data efficiency, long convergence process, and easy oscillation, so as to achieve the effect of ensuring learning effect, good performance effect, and shortening the learning cycle.
- Summary
- Abstract
- Description
- Claims
- Application Information
AI Technical Summary
Problems solved by technology
Method used
Image
Examples
Embodiment Construction
[0069] like image 3 As shown, the implementation steps of the continuous action online learning control method for automatic driving vehicles in this embodiment include:
[0070] 1) Get the current perceptual image I t ;
[0071] 2) Perceive the image I through the deep coding network t Encode to obtain the encoded state feature s t ;
[0072] 3) will encode the state feature s t Input the evaluator network (cerebellar model neural network value function network) and executor network (cerebellum model neural network policy network) of the actuator-evaluator model respectively, and both the evaluator network and the actuator network of the actuator-evaluator model use Cerebellum model neural network;
[0073] 4) Output action a through the actuator network t And the parameters of the actuator-evaluator model are updated through the evaluator network.
[0074] like Figure 4 As shown, the deep coding network adopted in step 2) is the HELM network model.
[0075] like ...
PUM
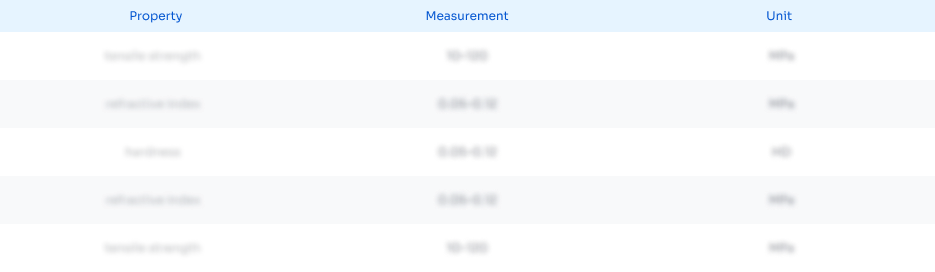
Abstract
Description
Claims
Application Information
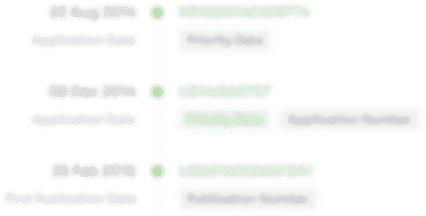
- R&D Engineer
- R&D Manager
- IP Professional
- Industry Leading Data Capabilities
- Powerful AI technology
- Patent DNA Extraction
Browse by: Latest US Patents, China's latest patents, Technical Efficacy Thesaurus, Application Domain, Technology Topic, Popular Technical Reports.
© 2024 PatSnap. All rights reserved.Legal|Privacy policy|Modern Slavery Act Transparency Statement|Sitemap|About US| Contact US: help@patsnap.com