Image denoising method based on residual convolutional self-coding network
A convolutional self-encoding and residual technology, applied in the field of deep learning, can solve the problems of only removing, not having generalization ability, easy to lose feature data, etc., and achieve high denoising quality and denoising accuracy
- Summary
- Abstract
- Description
- Claims
- Application Information
AI Technical Summary
Problems solved by technology
Method used
Image
Examples
Embodiment Construction
[0051] In order to efficiently remove the noise in the image, the residual convolution self-encoding block in this paper, such as figure 2 As shown, a multifunctional denoising residual convolutional autoencoder network model is built, such as image 3 shown. refer to figure 1 , shows a flowchart of the present invention, including the following steps.
[0052] Step 1. Preparation method of training set and data set:
[0053] The preprocessed original image and the corresponding noise-containing image are used as the training set and the test set, and the specific steps are as follows:
[0054] (1) Using the seismic data set of the Rock Mass Identification Challenge of Tomlinson Geophysical Services, the three-channel seismic image of 101*101 pixels is preprocessed into a single-channel grayscale image of 100*100 pixels;
[0055](2) Add corresponding noise to the preprocessed grayscale image, wherein, the training set is added with additive Gaussian noise, and the test se...
PUM
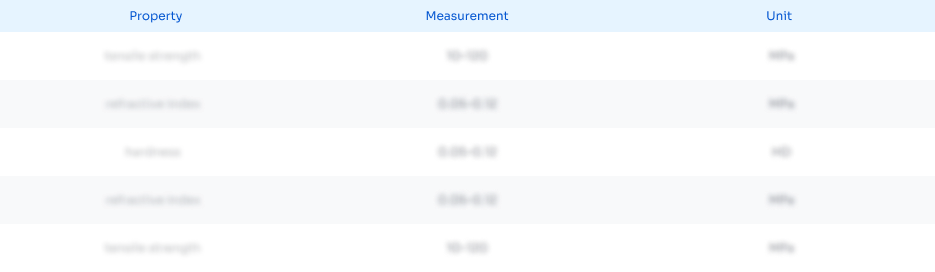
Abstract
Description
Claims
Application Information
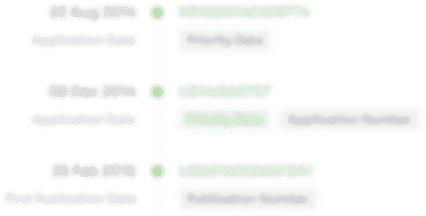
- R&D Engineer
- R&D Manager
- IP Professional
- Industry Leading Data Capabilities
- Powerful AI technology
- Patent DNA Extraction
Browse by: Latest US Patents, China's latest patents, Technical Efficacy Thesaurus, Application Domain, Technology Topic, Popular Technical Reports.
© 2024 PatSnap. All rights reserved.Legal|Privacy policy|Modern Slavery Act Transparency Statement|Sitemap|About US| Contact US: help@patsnap.com