Multi-agent cooperation framework based on deep reinforcement learning
A reinforcement learning and deep technology, applied in the field of virtual reality and artificial intelligence, can solve problems such as complexity, dimensionality disaster, and low learning efficiency, and achieve the effects of strong versatility, stability, and scalability
- Summary
- Abstract
- Description
- Claims
- Application Information
AI Technical Summary
Problems solved by technology
Method used
Image
Examples
Embodiment Construction
[0030] The present invention will be further described in detail below in conjunction with the accompanying drawings and specific embodiments.
[0031] Such as figure 1 As shown, the multi-agent collaboration framework based on deep reinforcement learning of the present invention consists of four parts, including: agent, billboard, Actor-Critic-based deep reinforcement learning module and next-moment state calculation module.
[0032] An agent is defined by its current state, velocity, and desired goal, where the current state is defined by the agent's current position p c and towards θ c Common representation; speed defines the moving rate and direction of the agent; the expected target provides the moving target point of the agent, and the expected moving direction can be calculated according to the current position and the target position.
[0033] The billboard is mainly responsible for the storage, update and transmission of information. The information stored in the bi...
PUM
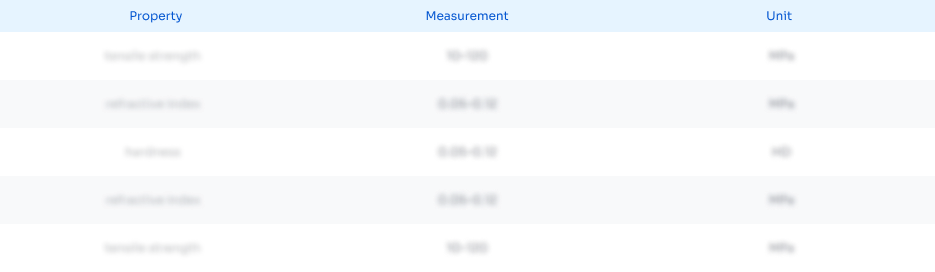
Abstract
Description
Claims
Application Information
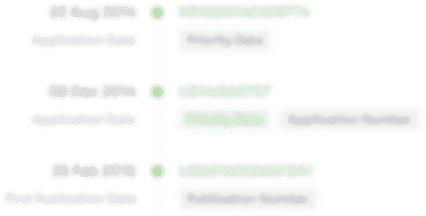
- R&D Engineer
- R&D Manager
- IP Professional
- Industry Leading Data Capabilities
- Powerful AI technology
- Patent DNA Extraction
Browse by: Latest US Patents, China's latest patents, Technical Efficacy Thesaurus, Application Domain, Technology Topic, Popular Technical Reports.
© 2024 PatSnap. All rights reserved.Legal|Privacy policy|Modern Slavery Act Transparency Statement|Sitemap|About US| Contact US: help@patsnap.com