Industrial process soft measurement method based on layer-by-layer data expansion deep learning
A deep learning and industrial process technology, applied in the field of soft measurement, can solve problems such as too few training samples and the inability of deep learning model technology to achieve satisfactory prediction accuracy.
- Summary
- Abstract
- Description
- Claims
- Application Information
AI Technical Summary
Problems solved by technology
Method used
Image
Examples
specific Embodiment 1
[0139] This embodiment shows the application of the technical solution of the present invention to the industrial process soft sensor of layer-by-layer data expansion and deep learning in the prediction of product quality in the hydrocracking process, including:
[0140] Based on the requirements of the production process, the 10% distillation point of jet fuel in the hydrocracking production process is selected as the output variable y, and 43 process variables that have a greater impact on the output variable are selected from the process through mechanism analysis as layer-by-layer data expansion deep learning The input variables of the model, as shown in Table 1, are recorded as x (1) ,x (2) ,...,x (43) . 600 data samples of the hydrocracking process were extracted as training data, and all variables were normalized.
[0141] Table 1 Selected 43 process variables that have a greater impact on the 10% distillation point of jet fuel
[0142]
[0143]
[0144]Constr...
specific Embodiment 2
[0173] This embodiment also shows that a method for predicting the quality of industrial process products of the present invention is applied to the prediction of product quality in the steel sintering process, including:
[0174] Based on the requirements of the production process, the content of ferrous oxide (FeO) is selected as the output variable y, and 19 process variables that have a great influence on the content of FeO are selected from the process through mechanism analysis as the input variables of the deep learning model for layer-by-layer data expansion, such as As shown in Table 3, denoted as x (1) ,x (2) ,...,x (19) . 1000 data samples of the steel sintering process were extracted as training data, and all variables were normalized.
[0175] Table 3 selects 19 process variables that have a greater impact on the content of ferrous oxide
[0176] Numbering Process variable description Numbering Process variable description 1 No. 1 bellows b...
PUM
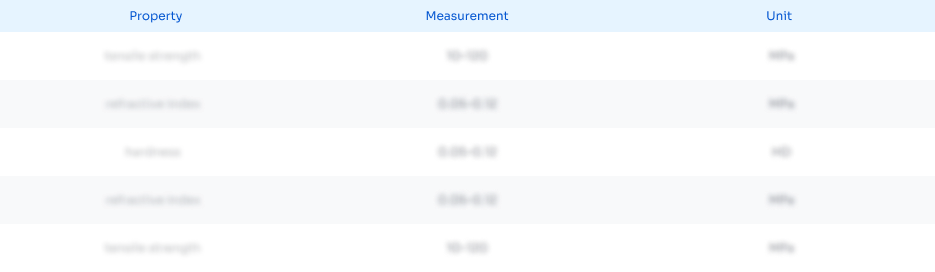
Abstract
Description
Claims
Application Information
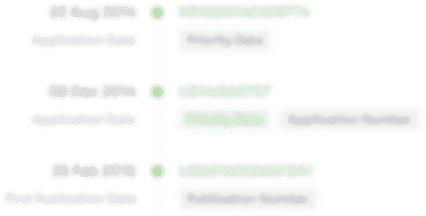
- R&D
- Intellectual Property
- Life Sciences
- Materials
- Tech Scout
- Unparalleled Data Quality
- Higher Quality Content
- 60% Fewer Hallucinations
Browse by: Latest US Patents, China's latest patents, Technical Efficacy Thesaurus, Application Domain, Technology Topic, Popular Technical Reports.
© 2025 PatSnap. All rights reserved.Legal|Privacy policy|Modern Slavery Act Transparency Statement|Sitemap|About US| Contact US: help@patsnap.com