Semi-supervised X-ray image automatic labeling based on generative adversarial network
A technology for image generation and automatic labeling, applied to biological neural network models, instruments, character and pattern recognition, etc., can solve problems such as model setting has a great influence, model prediction, and limited application range
- Summary
- Abstract
- Description
- Claims
- Application Information
AI Technical Summary
Problems solved by technology
Method used
Image
Examples
Embodiment Construction
[0056]Step 1: Build a network structure
[0057] On the basis of the generated confrontation network, the present invention proposes a semi-supervised X-ray image automatic labeling method based on the generated confrontation network, such as figure 1 shown. The generator sends random noise z to the generator network structure composed of multi-layer deconvolution, outputs the generated samples that fit the real data, and adds the generated image samples of the generator to the database image to guide the network training. For a K class For classification problems, use the newly generated class y=K+1 to annotate the generated image samples, and accordingly expand the dimension of the discriminator output softmax classifier from K to K+1:
[0058] (1) Build the generator:
[0059] The generator network structure uses a multi-layer deconvolution network to upsample the random noise vector z to generate a generated image of a specified size, such as figure 2 shown. First, th...
PUM
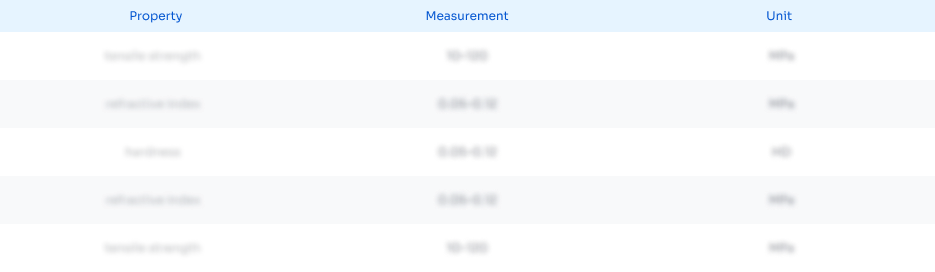
Abstract
Description
Claims
Application Information
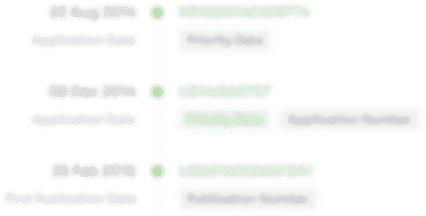
- R&D Engineer
- R&D Manager
- IP Professional
- Industry Leading Data Capabilities
- Powerful AI technology
- Patent DNA Extraction
Browse by: Latest US Patents, China's latest patents, Technical Efficacy Thesaurus, Application Domain, Technology Topic, Popular Technical Reports.
© 2024 PatSnap. All rights reserved.Legal|Privacy policy|Modern Slavery Act Transparency Statement|Sitemap|About US| Contact US: help@patsnap.com