Face beauty prediction method based on multi-task transfer learning
A technology of multi-task learning and transfer learning, applied in the field of face beauty prediction based on multi-task transfer learning, can solve the problems of small database size, prone to over-fitting, difficulty in deep network models, etc., and achieve good model generalization Ability to utilize and improve the effect of accuracy
- Summary
- Abstract
- Description
- Claims
- Application Information
AI Technical Summary
Problems solved by technology
Method used
Image
Examples
Embodiment Construction
[0041] The specific embodiment of the present invention will be further described below in conjunction with accompanying drawing:
[0042] Such as figure 1 As shown, the present invention provides a face beauty prediction method based on multi-task transfer learning. The present invention enhances the accuracy of face beauty prediction by adding expression recognition and age recognition; Fitting, and the computing equipment is not enough, this project intends to use mainstream VGG, ResNet, GoogleNet and other backbone deep convolutional networks as shared feature learning network structure, and through model migration, the trained convolutional network will be trained to transfer shared features. During the training process, network parameters are shared between each task, and shared features are learned, thereby improving the accuracy of the network for single-task learning. Specifically include the following steps:
[0043] S1), constructing multi-task learning face datab...
PUM
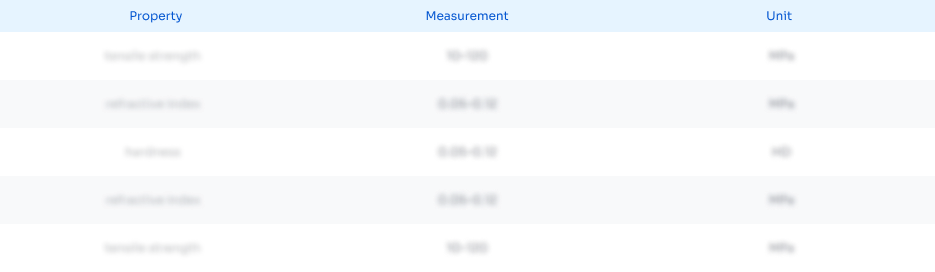
Abstract
Description
Claims
Application Information
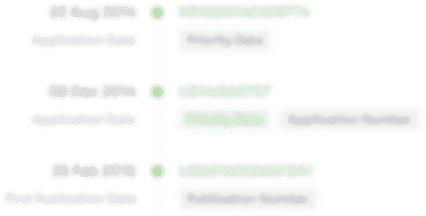
- R&D Engineer
- R&D Manager
- IP Professional
- Industry Leading Data Capabilities
- Powerful AI technology
- Patent DNA Extraction
Browse by: Latest US Patents, China's latest patents, Technical Efficacy Thesaurus, Application Domain, Technology Topic, Popular Technical Reports.
© 2024 PatSnap. All rights reserved.Legal|Privacy policy|Modern Slavery Act Transparency Statement|Sitemap|About US| Contact US: help@patsnap.com