High-order factor decomposer method based on sparse group Lasso
A factoring, high-level technology, applied in instruments, complex mathematical operations, calculations, etc., can solve problems such as the inability to directly use the prior information of the cross-term model, and achieve the effect of good performance, improved noise characteristics, and fewer parameters
- Summary
- Abstract
- Description
- Claims
- Application Information
AI Technical Summary
Problems solved by technology
Method used
Image
Examples
Embodiment Construction
[0035] 1. The special high-order cross structure of the high-order factorization machine is used, and the SGL regular term is used to punish the objective function, thereby realizing the structural sparseness of the feature, thereby removing noise and improving the performance of the model. The objective function of the new model is as follows:
[0036]
[0037] 2. Use the FOBOS algorithm to optimize the new objective function. The FOBOS algorithm is an iterative optimization algorithm. The specific process is as follows:
[0038] According to the FOBOS algorithm, formula (7) is first divided into two parts
[0039] f(ω,p (2) ,...,p (m) )+r(ω,p (2) ,...,p (m) ) (8)
[0040] in and The FOBOS update process is:
[0041] Step 1: Choose an appropriate regularization parameter β 1 and beta 2 , and the learning rate η, let time t=0.
[0042] Step 2: Yes Perform the standard gradient descent procedure.
[0043] Step 3: On the basis of Step 2, use the optimization al...
PUM
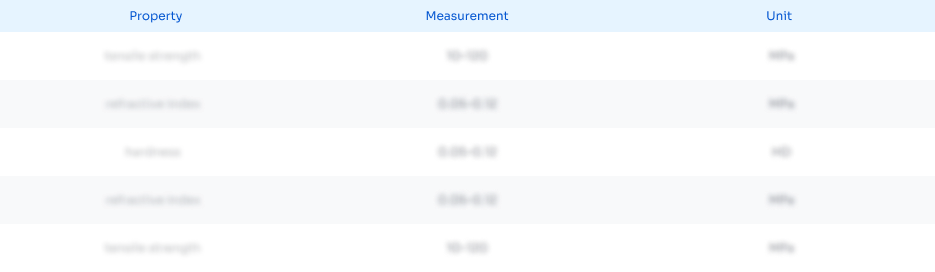
Abstract
Description
Claims
Application Information
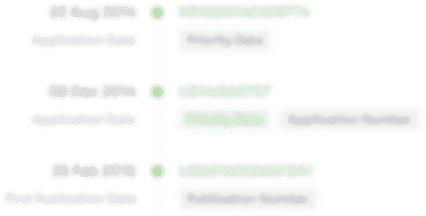
- R&D
- Intellectual Property
- Life Sciences
- Materials
- Tech Scout
- Unparalleled Data Quality
- Higher Quality Content
- 60% Fewer Hallucinations
Browse by: Latest US Patents, China's latest patents, Technical Efficacy Thesaurus, Application Domain, Technology Topic, Popular Technical Reports.
© 2025 PatSnap. All rights reserved.Legal|Privacy policy|Modern Slavery Act Transparency Statement|Sitemap|About US| Contact US: help@patsnap.com