Method for optimizing exception detection model based on deep learning
An anomaly detection and deep learning technology, applied in the field of machine learning, can solve the problems of multi-hours and labor cost preprocessing, high preprocessing cost, achieve the effect of achieving model generalization performance and solving the problem of imbalance between classes
- Summary
- Abstract
- Description
- Claims
- Application Information
AI Technical Summary
Problems solved by technology
Method used
Image
Examples
Embodiment 1
[0020] Example 1: Initial model training
[0021] (1) Basic sample preparation: Collect image data of production products in industrial production, with 1000 as the basic quantity.
[0022] (2) Training model: Based on the deep learning anomaly detection algorithm, train the anomaly detection model.
Embodiment 2
[0023] Embodiment 2: real-time detection
[0024] (1) Model detection: While inputting sample data in real time, model detection is performed, and the classification probability of the output sample is output.
[0025] (2) Calculate the effective sample probability interval: let the original data set, the number of positive samples be N P , the number of negative samples is N N , the number of predicted samples is P, and the number of wrong samples is P F , σ is the sigmoid function, so that the probability interval of the effective sample, that is, the fuzzy interval predicted by the model is: where parameters Defined as 0.5, the parameter β is defined as 0.5. The influencing factors in the formula are: the proportional coefficient of positive and negative samples in the original data set, when the class is unbalanced, the interval for judging negative samples should be increased; the accuracy of model detection, when the accuracy rate becomes higher, the range of fuzzy...
Embodiment 3
[0027] Embodiment 3: Judging whether the balance condition of the sample library is met
[0028] (1) Determine whether the sample library meets the requirements: the number of positive and negative samples satisfies N P N .
[0029] (2) Loop processing: When the sample library does not meet the requirements, the loop is started, model training is performed on the new sample library, and the anomaly detection model is iteratively optimized.
PUM
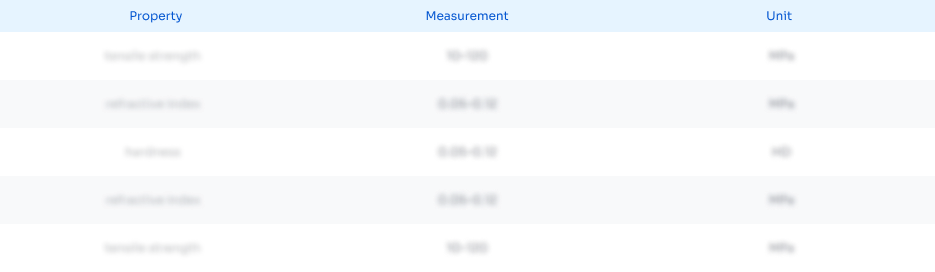
Abstract
Description
Claims
Application Information
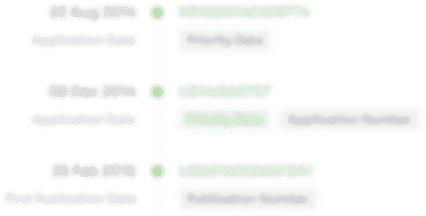
- Generate Ideas
- Intellectual Property
- Life Sciences
- Materials
- Tech Scout
- Unparalleled Data Quality
- Higher Quality Content
- 60% Fewer Hallucinations
Browse by: Latest US Patents, China's latest patents, Technical Efficacy Thesaurus, Application Domain, Technology Topic, Popular Technical Reports.
© 2025 PatSnap. All rights reserved.Legal|Privacy policy|Modern Slavery Act Transparency Statement|Sitemap|About US| Contact US: help@patsnap.com