Skeleton data behavior identification method based on graph convolutional neural network
A convolutional neural network and recognition method technology, applied in the fields of deep learning and behavior recognition, can solve the problems of ignoring the absolute motion and time information of skeleton joints, difficult to learn sequence time information, difficult to represent nonlinear mapping, etc.
- Summary
- Abstract
- Description
- Claims
- Application Information
AI Technical Summary
Problems solved by technology
Method used
Image
Examples
Embodiment Construction
[0017] Embodiments of the invention are described in detail below, examples of which are illustrated in the accompanying drawings. The embodiments described with reference to the figures are exemplary, and are intended to explain the present invention, and should not be construed as limiting the present invention.
[0018] Before introducing the behavior recognition method of skeleton data based on graph convolutional neural network, the data selected in this embodiment will be introduced first. Human skeleton data can be collected by depth sensors (such as Kinect). Currently, there are a large number of open source skeleton data sets, such as NTU RGB+D, SYSU-3D, HDM05, UT-Kinect, etc. Among them, the NTU RGB-D dataset is currently the largest skeleton-based action recognition dataset, with more than 56,000 sequences and 4 million frames, a total of 60 types of actions, and each skeleton has 25 joint points, involving single-person actions and double-person actions . This em...
PUM
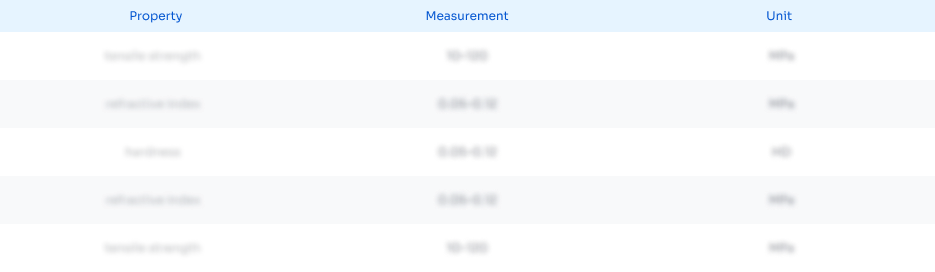
Abstract
Description
Claims
Application Information
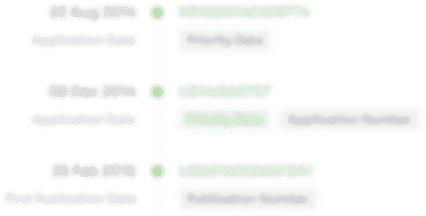
- R&D Engineer
- R&D Manager
- IP Professional
- Industry Leading Data Capabilities
- Powerful AI technology
- Patent DNA Extraction
Browse by: Latest US Patents, China's latest patents, Technical Efficacy Thesaurus, Application Domain, Technology Topic.
© 2024 PatSnap. All rights reserved.Legal|Privacy policy|Modern Slavery Act Transparency Statement|Sitemap