Adversarial sample generation method based on Bayesian optimization
An anti-sample and iterative technology, applied to biological neural network models, instruments, platform integrity maintenance, etc., can solve problems such as large query overhead, and achieve the effect of reducing impact
- Summary
- Abstract
- Description
- Claims
- Application Information
AI Technical Summary
Problems solved by technology
Method used
Image
Examples
Embodiment Construction
[0027] The present invention takes an original image as input, calculates the structural similarity between the original image and the random Gaussian image, calculates its gradient, and selects the dimension corresponding to the smallest gradient value. Use Bayesian optimization dimension by dimension to get the best perturbation value. The disturbances obtained by multiple iterations are added together until the category prediction result of the DNN classifier is changed.
[0028] The following examples illustrate the specific implementation of the entire process of the present invention as follows (see the effect diagram of each step figure 2 ):
[0029] Step 1: Obtain the true category y of the source image x c And its probability M c
[0030] x is the original image vector (e.g. figure 1 Shown), Δx is an all-zero disturbance vector with the same dimension as x, x G Is a random vector sampled from a Gaussian distribution with the same dimension as x (such as figure 2 Shown). ...
PUM
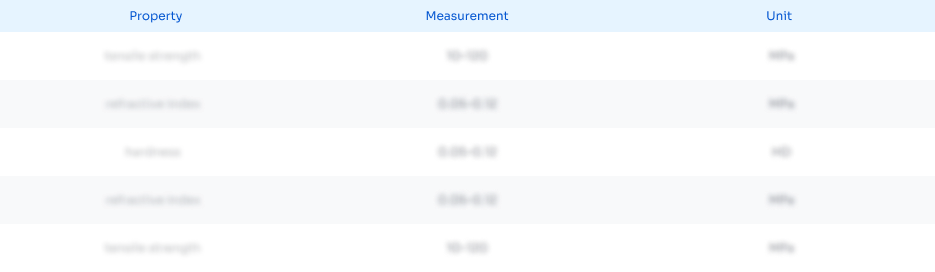
Abstract
Description
Claims
Application Information
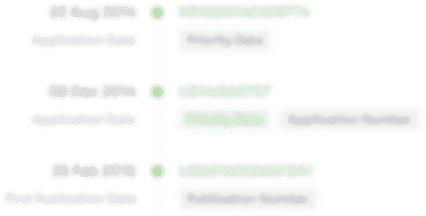
- R&D
- Intellectual Property
- Life Sciences
- Materials
- Tech Scout
- Unparalleled Data Quality
- Higher Quality Content
- 60% Fewer Hallucinations
Browse by: Latest US Patents, China's latest patents, Technical Efficacy Thesaurus, Application Domain, Technology Topic, Popular Technical Reports.
© 2025 PatSnap. All rights reserved.Legal|Privacy policy|Modern Slavery Act Transparency Statement|Sitemap|About US| Contact US: help@patsnap.com