Method for reducing distributed machine learning communication overhead
A technology of machine learning and communication overhead, applied in machine learning, instrumentation, resource allocation, etc., can solve problems such as prolonging communication time, and achieve the effect of reducing communication traffic and reducing communication overhead
- Summary
- Abstract
- Description
- Claims
- Application Information
AI Technical Summary
Problems solved by technology
Method used
Image
Examples
Embodiment Construction
[0030] Below in conjunction with specific embodiment, further illustrate the present invention, should be understood that these embodiments are only used to illustrate the present invention and are not intended to limit the scope of the present invention, after having read the present invention, those skilled in the art will understand various equivalent forms of the present invention All modifications fall within the scope defined by the appended claims of the present application.
[0031] The method for reducing the communication overhead of distributed machine learning provided by the present invention can be applied to the fields of image classification, text classification, etc., and is suitable for scenarios where there are a large number of data sets to be classified and a large number of machine learning model parameters are used. Taking the image classification application as an example, in the method of the present invention, the training image data will be distribute...
PUM
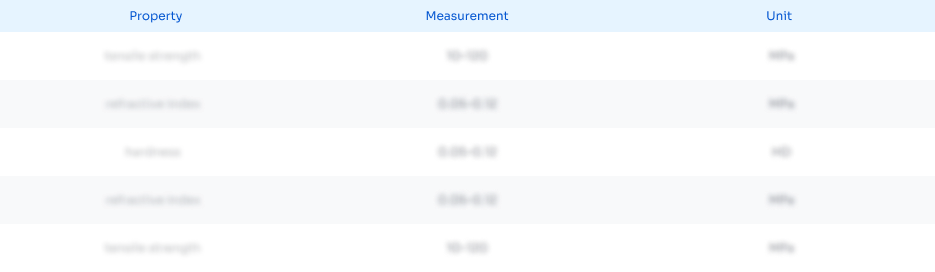
Abstract
Description
Claims
Application Information
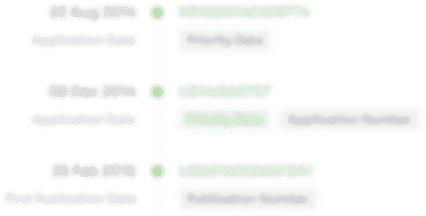
- Generate Ideas
- Intellectual Property
- Life Sciences
- Materials
- Tech Scout
- Unparalleled Data Quality
- Higher Quality Content
- 60% Fewer Hallucinations
Browse by: Latest US Patents, China's latest patents, Technical Efficacy Thesaurus, Application Domain, Technology Topic, Popular Technical Reports.
© 2025 PatSnap. All rights reserved.Legal|Privacy policy|Modern Slavery Act Transparency Statement|Sitemap|About US| Contact US: help@patsnap.com