Fuzzy integrated classifier with high interpretability based on parallel learning
An ensemble classifier and interpretability technology, applied in the fields of fuzzy ensemble fast classification, fuzzy recognition and machine learning based on parallel learning, which can solve problems such as heavy computational burden
- Summary
- Abstract
- Description
- Claims
- Application Information
AI Technical Summary
Problems solved by technology
Method used
Image
Examples
Embodiment Construction
[0026] A kind of fuzzy integrated classifier with high interpretability based on parallel learning of the present invention comprises the following steps in turn:
[0027] To achieve the above object, the present invention comprises the following steps in turn:
[0028] a) Quick construction method of zero-order TSK fuzzy classifier:
[0029] For a given dataset, the lth subset of training data and its corresponding label set where x i ∈R d ,y i ∈R, i=1,2,...,N l , the number of fuzzy rules K l , the regularization parameter C; specifically includes the following steps:
[0030] S1. Construct 5 Gaussian membership functions, whose central point set is {0, 0.25, 0.5, 0.75, 1}, randomly assign a value from the central point set in each dimension and construct a regular combination matrix
[0031] S2. Construct the kernel width matrix by assigning each element a random positive number
[0032] S3. Combine the matrix, the kernel width matrix and the above five Gauss...
PUM
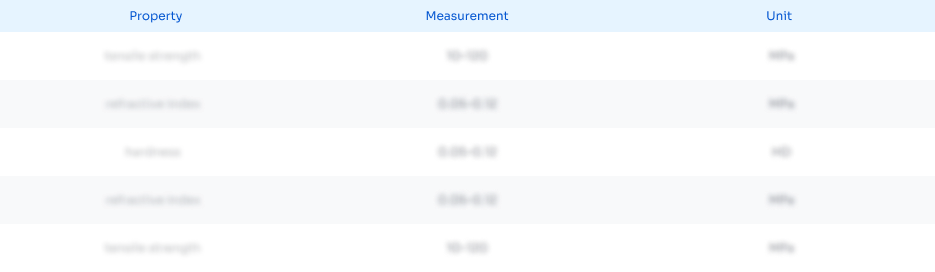
Abstract
Description
Claims
Application Information
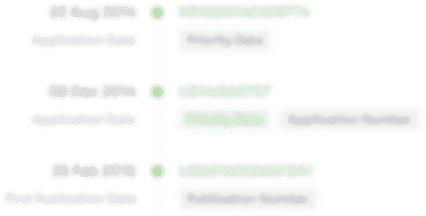
- R&D
- Intellectual Property
- Life Sciences
- Materials
- Tech Scout
- Unparalleled Data Quality
- Higher Quality Content
- 60% Fewer Hallucinations
Browse by: Latest US Patents, China's latest patents, Technical Efficacy Thesaurus, Application Domain, Technology Topic, Popular Technical Reports.
© 2025 PatSnap. All rights reserved.Legal|Privacy policy|Modern Slavery Act Transparency Statement|Sitemap|About US| Contact US: help@patsnap.com