Image recognition method, device and equipment based on lightweight network model
A network model and image recognition technology, applied in the field of pattern recognition, can solve the problems of low accuracy of wide network, dependence on expensive hardware configuration and time-consuming calculation and training
- Summary
- Abstract
- Description
- Claims
- Application Information
AI Technical Summary
Problems solved by technology
Method used
Image
Examples
Embodiment 1
[0071] Such as Figure 1 to Figure 3 As shown, this embodiment provides an image recognition method based on a lightweight network model, which specifically includes the following steps:
[0072] S1, acquiring a target image to be identified;
[0073] S2, inputting the target image into the trained lightweight network model;
[0074] S3, using the trained lightweight network model to classify the target image.
[0075] Wherein, the process of obtaining the lightweight network model includes the following steps:
[0076] S21, according to the construction method of the convolutional neural network, construct a variant convolutional neural network (CNN) without a fully connected layer, the variant convolutional neural network includes one or more network layers, and the network layer includes a convolutional layers and a pooling layer;
[0077] S22, input the marked image to be classified; the marked image to be classified obtains the feature of the image through the variant...
Embodiment 2
[0142] The image recognition method based on the lightweight network model described in Embodiment 1 can be widely used in the field of image recognition. In this embodiment, the MNIST data set is used to test and train the network. The detailed process is as follows:
[0143] Construct a variant convolutional neural network (CNN) to extract image features. Construct a variant CNN based on the LeNet network structure. In the variant convolutional neural network, the first convolutional layer, the first pooling layer, the second convolutional layer, and the second pooling layer are connected in sequence, and the first convolutional layer The size of the convolution filter and the second convolutional layer are both 5*5, and the moving step is 1, where the depth of the filter of the first convolutional layer is 32, and the depth of the filter of the second convolutional layer is 64; the first pooling layer and the second pooling layer both use the maximum pooling method, and the...
Embodiment 3
[0174] Corresponding to the above method embodiment, this embodiment also provides an image recognition device based on a lightweight network model, the image recognition device based on a lightweight network model described below and the image recognition device based on a lightweight network model described above The identification methods can be referred to in correspondence with each other.
[0175] see Figure 5 As shown, the device includes the following modules: target image acquisition module 101, target image input module 102, classification recognition module 103 and target model acquisition module 104;
[0176] Wherein, the target image acquisition module 101 is used to acquire the target image to be identified;
[0177] The target image input module 102 is used to input the target image into the target lightweight network model; the lightweight network model is obtained by removing the variant convolutional neural network and the width network structure of the ful...
PUM
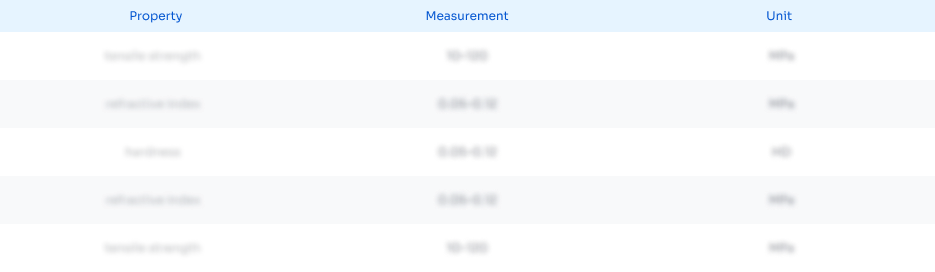
Abstract
Description
Claims
Application Information
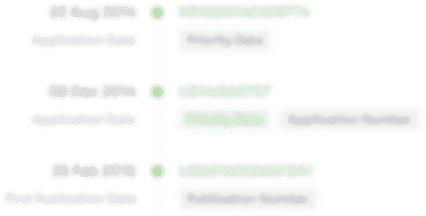
- R&D Engineer
- R&D Manager
- IP Professional
- Industry Leading Data Capabilities
- Powerful AI technology
- Patent DNA Extraction
Browse by: Latest US Patents, China's latest patents, Technical Efficacy Thesaurus, Application Domain, Technology Topic, Popular Technical Reports.
© 2024 PatSnap. All rights reserved.Legal|Privacy policy|Modern Slavery Act Transparency Statement|Sitemap|About US| Contact US: help@patsnap.com