Image restoration method based on low-rank tensor completion and discrete total variation
A restoration method and a total variation technology, applied in the field of image processing, can solve problems such as long restoration time and reduced algorithm efficiency
- Summary
- Abstract
- Description
- Claims
- Application Information
AI Technical Summary
Problems solved by technology
Method used
Image
Examples
Embodiment 1
[0183] The image inpainting method based on low-rank tensor completion and discrete total variation of this application is shown in the algorithm as:
[0184] Input: incomplete tensor Initialize the number of iterations T=0, the maximum number of iterations b, λ, ρ 1 , ρ 2 , ρ 3 and μ ∈ [1, 1.5];
[0185] output: restored tensor
[0186] S6.1, initialization
[0187] S6.2. If T≤b, continue to execute downward; otherwise, output recovery tensor
[0188] S6.3, through formulas (9), (11), (18), (20) to update respectively
[0189] S6.4, update
[0190] S6.5, update
[0191] S6.6, update
[0192] S6.7. Calculate ρ 1 =μρ 1 ,ρ 2 =μρ 2 ,ρ 3 =μρ 3 ;
[0193] S6.8, T=T+1, and return to S6.2.
[0194] S6.1 in the algorithm corresponds to steps 1-3 in the image restoration method, S6.3-S6.7 in the algorithm corresponds to step 4 in the image restoration method, and S6.2, S6. 8 corresponds to step 5 in the image restoration method.
[0195] It should be n...
PUM
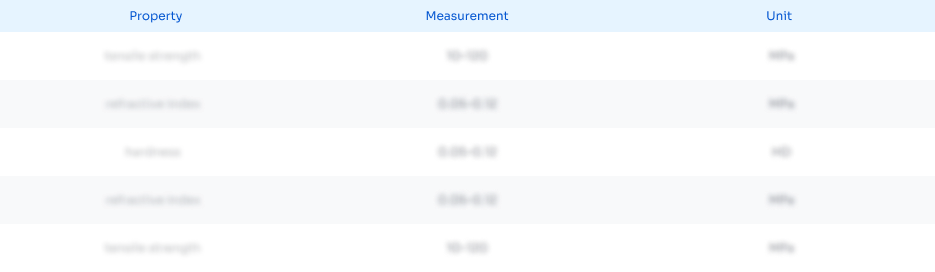
Abstract
Description
Claims
Application Information
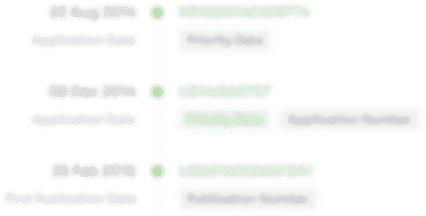
- R&D Engineer
- R&D Manager
- IP Professional
- Industry Leading Data Capabilities
- Powerful AI technology
- Patent DNA Extraction
Browse by: Latest US Patents, China's latest patents, Technical Efficacy Thesaurus, Application Domain, Technology Topic, Popular Technical Reports.
© 2024 PatSnap. All rights reserved.Legal|Privacy policy|Modern Slavery Act Transparency Statement|Sitemap|About US| Contact US: help@patsnap.com