Sand storm level prediction method based on Stacking integration strategy
A technology that integrates strategies and prediction methods, applied in the computer field, can solve problems such as difficult generalization, large amount of meteorological data, and difficulty in fitting neural networks, and achieve good generalization, prediction and classification performance.
- Summary
- Abstract
- Description
- Claims
- Application Information
AI Technical Summary
Problems solved by technology
Method used
Image
Examples
Embodiment Construction
[0068] The implementation of the present invention will be described in detail below in conjunction with the drawings and examples.
[0069] Recurrent Neural Network ((Recurrent Neural Network, RNN) is a kind of in deep learning model. This type of neural network is usually used for processing sequence data. Due to the spatiotemporal characteristics and periodicity that meteorological data have, therefore, the present invention will adopt recurrent neural network The network is used as a first-level classifier, and the Gated Recursive Unit (GRU) is used to solve the long-term dependence problems in the traditional RNN, and analyze and predict the collected sandstorm meteorological sequence data.
[0070] Convolutional Neural Network (CNN) generally has better results in feature extraction of high-dimensional data. Convolutional neural network is a kind of neural network specially used to process data with similar grid structure. Due to its strong feature extraction ability, it...
PUM
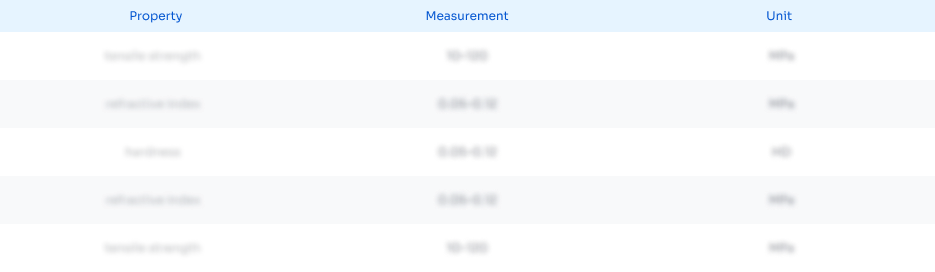
Abstract
Description
Claims
Application Information
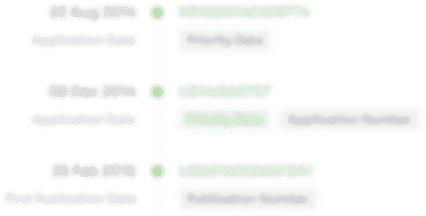
- R&D
- Intellectual Property
- Life Sciences
- Materials
- Tech Scout
- Unparalleled Data Quality
- Higher Quality Content
- 60% Fewer Hallucinations
Browse by: Latest US Patents, China's latest patents, Technical Efficacy Thesaurus, Application Domain, Technology Topic, Popular Technical Reports.
© 2025 PatSnap. All rights reserved.Legal|Privacy policy|Modern Slavery Act Transparency Statement|Sitemap|About US| Contact US: help@patsnap.com