Multi-view subspace clustering method based on joint subspace learning
A technology of subspace learning and clustering methods, applied in the direction of instruments, character and pattern recognition, computer components, etc., can solve problems that affect the clustering effect
- Summary
- Abstract
- Description
- Claims
- Application Information
AI Technical Summary
Problems solved by technology
Method used
Image
Examples
Embodiment 1
[0073] A multi-view subspace clustering method based on joint subspace learning, such as figure 1 shown, including the following steps:
[0074] S1. Obtain the multi-view feature matrix of the original data set;
[0075] For each multi-view image in the original data set, different types of data features are extracted respectively, the data features of the same type are formed into the feature matrix of this view, and the feature matrices of all views are formed into the multi-view feature matrix of the original data set in Represents the vth view feature matrix in the original dataset, m v Indicates the dimension of the data features of the vth view, and n indicates the number of data features of the vth view; where V≥2, indicates the number of multi-views.
[0076] S2. Construct the objective function of multi-view subspace clustering based on joint subspace learning;
[0077] S2.1. Perform joint subspace learning on multi-view data features, and find the low-dimension...
Embodiment 2
[0122] A multi-view subspace clustering method based on joint subspace learning, such as figure 1 shown, including the following steps:
[0123] S1. Obtain the multi-view feature matrix of the original dataset
[0124] In this embodiment, the original data set used is the ORL face data set. The ORL face dataset contains a total of 400 face images from 40 people, each with 10 images.
[0125] For the multi-view images of ORL face dataset, the features of three views are extracted Includes: gray value features LBP characteristics and Gabor wavelets feature
[0126] S2. Construct the objective function of multi-view subspace clustering based on joint subspace learning;
[0127]
[0128] s.t.P (v)T x (v) =P (v)T x (v) Z+E (v) ,P (v)T P (v) =I,‖Z‖ 0 ≤T 0 , v∈[1,2,3]
[0129] Wherein in this embodiment 2, the balance factor α=0.7 is set, and the dimension d=150 of the low-dimensional embedding space;
[0130] S3. Using an alternate optimization method to s...
PUM
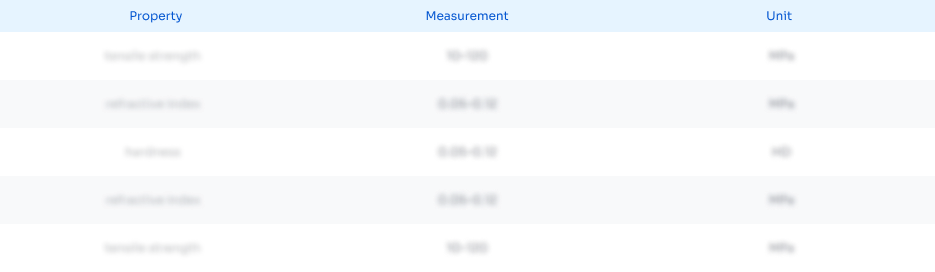
Abstract
Description
Claims
Application Information
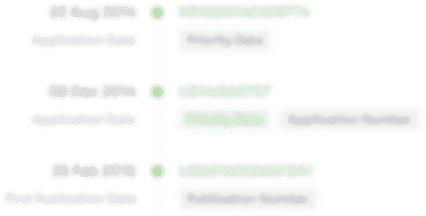
- R&D Engineer
- R&D Manager
- IP Professional
- Industry Leading Data Capabilities
- Powerful AI technology
- Patent DNA Extraction
Browse by: Latest US Patents, China's latest patents, Technical Efficacy Thesaurus, Application Domain, Technology Topic, Popular Technical Reports.
© 2024 PatSnap. All rights reserved.Legal|Privacy policy|Modern Slavery Act Transparency Statement|Sitemap|About US| Contact US: help@patsnap.com