Semi-supervised multi-mark distance metric learning method fusing local metric
A technology of distance measurement and learning method, which is applied in the field of multi-label learning scenarios, can solve the problem of less feature space processing, reduce the cost of human labeling, and promote the effect of practical application
- Summary
- Abstract
- Description
- Claims
- Application Information
AI Technical Summary
Problems solved by technology
Method used
Image
Examples
Embodiment Construction
[0036] The present invention will be described in further detail below in conjunction with the accompanying drawings.
[0037] Such as figure 1 As shown in , a semi-supervised multi-label distance metric learning method that fuses local metrics includes the following steps:
[0038] 1. Sampling any multi-label application scenarios such as images, videos, texts, etc. to obtain training data, extract corresponding features and manually label a small number of examples to obtain training data D=L∪U={(x i ,Y i )|1≤i≤n}∪{x j |1≤j≤m}, where Y i Label vectors for q-dimensions.
[0039] 2. Preprocess the training data. For the labeled data L, filter out the samples whose label occupancy rate is less than the set threshold. For the unlabeled data U, remove abnormal points through clustering and other operations to improve the sample quality.
[0040] 3. Based on the characteristics of multi-label data, the distance measure to be learned is expressed as a combined distance measure...
PUM
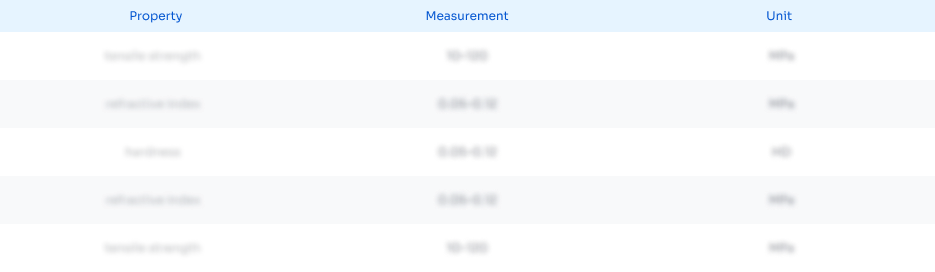
Abstract
Description
Claims
Application Information
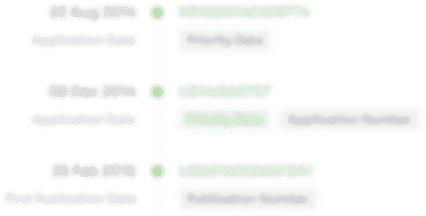
- R&D Engineer
- R&D Manager
- IP Professional
- Industry Leading Data Capabilities
- Powerful AI technology
- Patent DNA Extraction
Browse by: Latest US Patents, China's latest patents, Technical Efficacy Thesaurus, Application Domain, Technology Topic, Popular Technical Reports.
© 2024 PatSnap. All rights reserved.Legal|Privacy policy|Modern Slavery Act Transparency Statement|Sitemap|About US| Contact US: help@patsnap.com